Spectral super-resolution adaptive weighted attention mechanism deep network data processing method
An adaptive weighting, deep network technology, applied in the field of hyperspectral image processing, can solve the problems of lack of correlation, limiting the performance of super-resolution methods, ignoring the prior information of the camera response curve, etc., to achieve the effect of enhancing the ability of feature expression
- Summary
- Abstract
- Description
- Claims
- Application Information
AI Technical Summary
Problems solved by technology
Method used
Image
Examples
Embodiment Construction
[0064] In order to make the object, technical solution and advantages of the present invention more clear, the present invention will be further described in detail below in conjunction with the examples. It should be understood that the specific embodiments described here are only used to explain the present invention, not to limit the present invention.
[0065] Aiming at the problems existing in the prior art, the present invention provides a spectral super-resolution adaptive weighted attention mechanism deep network data processing method. The present invention will be described in detail below in conjunction with the accompanying drawings.
[0066] Such as figure 1 As shown, the spectral super-resolution adaptive weighted attention mechanism deep network data processing method provided by the present invention comprises the following steps:
[0067] S101: Select two spectral reconstruction challenge datasets for verification;
[0068] S102: Selection of evaluation inde...
PUM
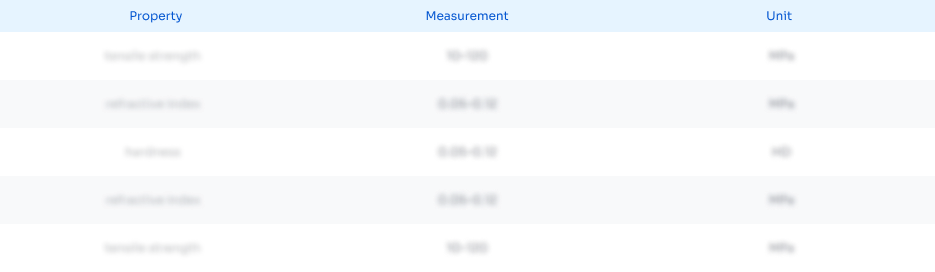
Abstract
Description
Claims
Application Information
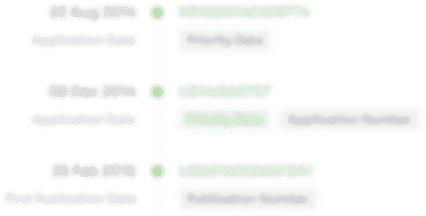
- R&D Engineer
- R&D Manager
- IP Professional
- Industry Leading Data Capabilities
- Powerful AI technology
- Patent DNA Extraction
Browse by: Latest US Patents, China's latest patents, Technical Efficacy Thesaurus, Application Domain, Technology Topic, Popular Technical Reports.
© 2024 PatSnap. All rights reserved.Legal|Privacy policy|Modern Slavery Act Transparency Statement|Sitemap|About US| Contact US: help@patsnap.com