Miniature neural network model
A neural network model and miniature technology, applied in the field of convolution group, can solve the problems of insufficient depth of convolution, too many channels, too many convolution kernels, etc. The effect of force and memory requirements
- Summary
- Abstract
- Description
- Claims
- Application Information
AI Technical Summary
Problems solved by technology
Method used
Image
Examples
Embodiment 1
[0025] S1. After preliminary extraction of the Core convolution kernel, multiple feature maps are generated. The Core convolution group in S1 contains 3×3 convolution kernels. The number of 3×3 convolution kernels in the Core convolution group is higher than that of the normal convolution group. The number is reduced, the number of channels of the input feature map of the 3×3 convolution kernel is reduced compared with the number of normal convolution groups, and it is effective by reducing the number of 3×3 convolution kernels and reducing the number of channels of the input feature map of the 3×3 convolution kernel It avoids the traditional insufficient convolution depth and too many convolution kernels, which lead to too many channels of the output feature map and a large number of parameters, which reduces the hardware computing power and memory requirements. The Core convolution group has a total of It consists of 10 Core convolution kernels and they are respectively Core4...
Embodiment 2
[0031] S1. After preliminary extraction of the Core convolution kernel, multiple feature maps are generated. The Core convolution group in S1 contains 3×3 convolution kernels. The number of 3×3 convolution kernels in the Core convolution group is higher than that of the normal convolution group. The number is reduced, the number of channels of the input feature map of the 3×3 convolution kernel is reduced compared with the number of normal convolution groups, and it is effective by reducing the number of 3×3 convolution kernels and reducing the number of channels of the input feature map of the 3×3 convolution kernel It avoids the traditional insufficient convolution depth and too many convolution kernels, which lead to too many channels of the output feature map and a large number of parameters, which reduces the hardware computing power and memory requirements. The Core convolution group has a total of It consists of 10 Core convolution kernels and they are respectively Core8...
Embodiment 3
[0037] S1. After preliminary extraction of the Core convolution kernel, multiple feature maps are generated. The Core convolution group in S1 contains 3×3 convolution kernels. The number of 3×3 convolution kernels in the Core convolution group is higher than that of the normal convolution group. The number is reduced, the number of channels of the input feature map of the 3×3 convolution kernel is reduced compared with the number of normal convolution groups, and it is effective by reducing the number of 3×3 convolution kernels and reducing the number of channels of the input feature map of the 3×3 convolution kernel It avoids the traditional insufficient convolution depth and too many convolution kernels, resulting in too many channels of the output feature map and a large number of parameters, which reduces the hardware computing power and memory requirements. The Core convolution group has a total of It consists of 10 Core convolution kernels and they are respectively Core9....
PUM
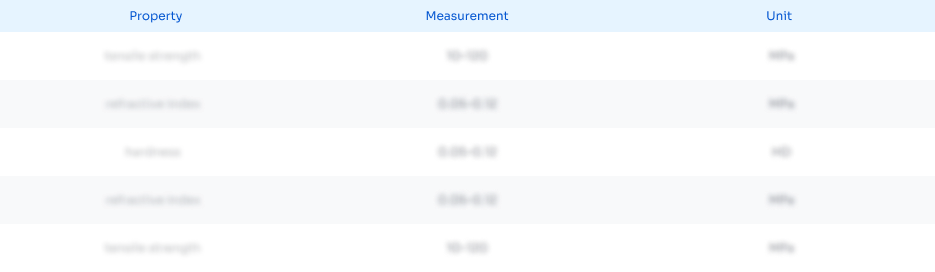
Abstract
Description
Claims
Application Information
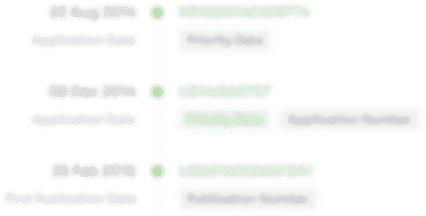
- R&D
- Intellectual Property
- Life Sciences
- Materials
- Tech Scout
- Unparalleled Data Quality
- Higher Quality Content
- 60% Fewer Hallucinations
Browse by: Latest US Patents, China's latest patents, Technical Efficacy Thesaurus, Application Domain, Technology Topic, Popular Technical Reports.
© 2025 PatSnap. All rights reserved.Legal|Privacy policy|Modern Slavery Act Transparency Statement|Sitemap|About US| Contact US: help@patsnap.com