A Printing Defect Detection Method Based on Deep Convolutional Generative Networks
A technology for printing defects and detection methods, applied in image analysis, image enhancement, instruments, etc., can solve problems such as the inability to accurately detect small defects, and achieve the effect of improving detection accuracy
Active Publication Date: 2022-05-31
HARBIN UNIV OF SCI & TECH
View PDF9 Cites 0 Cited by
- Summary
- Abstract
- Description
- Claims
- Application Information
AI Technical Summary
Problems solved by technology
However, at present, only large-area printing defects can be accurately detected, and accurate detection of small defects cannot be realized.
Method used
the structure of the environmentally friendly knitted fabric provided by the present invention; figure 2 Flow chart of the yarn wrapping machine for environmentally friendly knitted fabrics and storage devices; image 3 Is the parameter map of the yarn covering machine
View moreImage
Smart Image Click on the blue labels to locate them in the text.
Smart ImageViewing Examples
Examples
Experimental program
Comparison scheme
Effect test
Embodiment 1
[0053] S13, crop the picture: crop the picture, and the size is 512×512.
[0062]
[0064] S4, training the model, to obtain the generator that is successfully trained. The loss function formula is as follows:
[0065] loss (x
[0066] Where i is the training sample, x is the expected class probability, y is the class probability predicted by the model, and w is the weight.
the structure of the environmentally friendly knitted fabric provided by the present invention; figure 2 Flow chart of the yarn wrapping machine for environmentally friendly knitted fabrics and storage devices; image 3 Is the parameter map of the yarn covering machine
Login to View More PUM
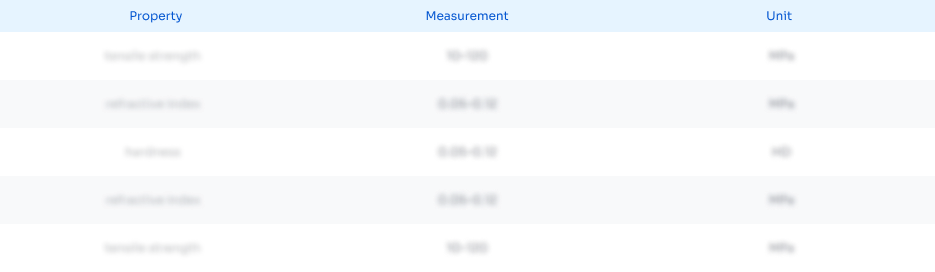
Abstract
A printing defect detection method based on deep convolution generation network, the invention relates to the problem of accurate detection of tiny defects in printed matter in defect detection technology. In recent years, deep learning has been widely used in defect detection. Although current methods have made progress on defect detection tasks in simple backgrounds, they still cannot accurately detect subtle defects. To solve this problem, the present invention proposes a printing defect detection method based on a deep convolutional network. First, add an upsampling module to reduce the loss in upsampling. Second, a self-attention mechanism is proposed, so that the network can generate images with more complex structures and more accurate details. Finally, the fitting generator generates the noise distribution of the image, removes the noise, and obtains the defect image. On the basis of ensuring the accuracy rate, the detection precision is improved. The invention is applied to the unsupervised detection of small printing defects.
Description
A printing defect detection method based on deep convolutional generative network technical field The present invention relates to the detection of tiny defects in printed matter Background technique [0002] With the improvement of living standards, people also have higher requirements for the appearance quality of printed matter. China owns In the huge printing market, printing inspection has also become a research hotspot in the academic circle. Traditional manual inspection is not only time-consuming and labor-intensive, Moreover, different quality inspectors have different detection standards, and at the same time, they are also accompanied by false detection and missed detection caused by fatigue. chasing this way The pursuit of quality and efficiency is gradually being neglected and replaced by automated machine vision inspection. Automated detection can Achieving 24-hour inspection at the same standard and speed, without the risk of artificial fatigue, and ...
Claims
the structure of the environmentally friendly knitted fabric provided by the present invention; figure 2 Flow chart of the yarn wrapping machine for environmentally friendly knitted fabrics and storage devices; image 3 Is the parameter map of the yarn covering machine
Login to View More Application Information
Patent Timeline
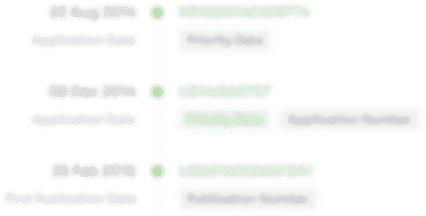
Patent Type & Authority Patents(China)
IPC IPC(8): G06T7/00G06T7/136
CPCG06T7/0002G06T7/136G06T2207/20081G06T2207/20084Y02P90/30
Inventor 何勇军高玉栋陈德运
Owner HARBIN UNIV OF SCI & TECH
Who we serve
- R&D Engineer
- R&D Manager
- IP Professional
Why Patsnap Eureka
- Industry Leading Data Capabilities
- Powerful AI technology
- Patent DNA Extraction
Social media
Patsnap Eureka Blog
Learn More Browse by: Latest US Patents, China's latest patents, Technical Efficacy Thesaurus, Application Domain, Technology Topic, Popular Technical Reports.
© 2024 PatSnap. All rights reserved.Legal|Privacy policy|Modern Slavery Act Transparency Statement|Sitemap|About US| Contact US: help@patsnap.com