Chapter-level neural machine translation method and system based on routing algorithm
A machine translation and text technology, applied in neural architecture, natural language translation, biological neural network models, etc., can solve the problems of identifying useful information, difficulty, and model modeling, etc., to improve quality and effect Effect
- Summary
- Abstract
- Description
- Claims
- Application Information
AI Technical Summary
Problems solved by technology
Method used
Image
Examples
Embodiment Construction
[0066] The present invention will be further described below in conjunction with the accompanying drawings and specific embodiments.
[0067] like figure 1 As shown, a routing algorithm-based article-level neural machine translation method includes the following steps:
[0068] Step 1: Input the context sentence and encode to generate encoded information. specifically:
[0069] Step 1.1: Through the embedding layer, convert the context sentence into its word vector representation sequence;
[0070] Step 1.2: Encode the context sentence through the encoder, and output the respective encoding representations of the previous sentence and the latter sentence.
[0071] Step 2: Input the source language sentence, and through the routing algorithm layer, fuse with the context encoding information to generate chapter-level information. specifically:
[0072] Step 2.1: Through the embedding layer, convert the source language sentence into its word vector representation sequence; ...
PUM
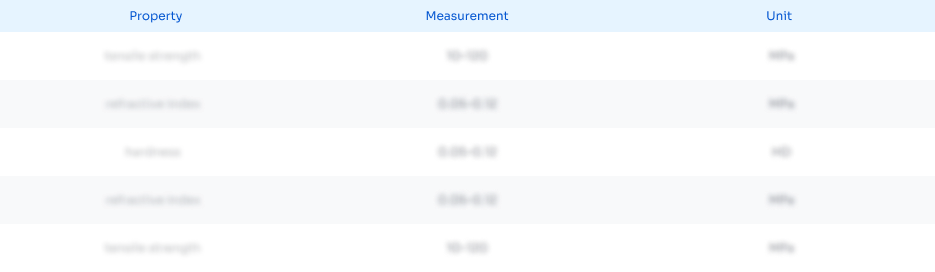
Abstract
Description
Claims
Application Information
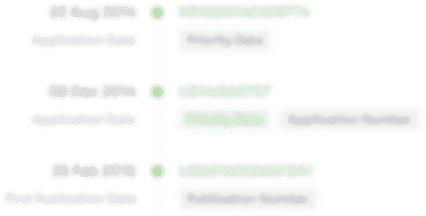
- R&D Engineer
- R&D Manager
- IP Professional
- Industry Leading Data Capabilities
- Powerful AI technology
- Patent DNA Extraction
Browse by: Latest US Patents, China's latest patents, Technical Efficacy Thesaurus, Application Domain, Technology Topic.
© 2024 PatSnap. All rights reserved.Legal|Privacy policy|Modern Slavery Act Transparency Statement|Sitemap