Federal model training method and device, electronic equipment and storage medium
A model training and federation technology, which is applied in multi-programming devices, program control design, electrical digital data processing, etc., can solve the problems of the federation model failing to converge, and achieve the goal of avoiding failure to converge, improving performance, and improving network resource utilization. Effect
- Summary
- Abstract
- Description
- Claims
- Application Information
AI Technical Summary
Benefits of technology
Problems solved by technology
Method used
Image
Examples
Embodiment 1
[0029] figure 1 It is a flowchart of a federated model training method provided in Embodiment 1 of the present invention. This embodiment is applicable to the case of federated model training in an edge computing network, and the method can be executed by the federated model training device provided in the embodiment of the present invention. , the device may be implemented by software and / or hardware, and typically, the device may be integrated into a server in the federated model training system.
[0030] see further figure 2 , figure 2 A logical architecture diagram of a federated model training system is provided for an embodiment of the present invention. The federated model training system provided in this embodiment includes at least one parameter server and multiple edge devices (ie clients, also called edge working nodes). The parameter server is used to communicate with the client through the wireless network to transmit model parameters; the client is used to tr...
Embodiment 2
[0098] Figure 5 It is a schematic structural diagram of a federated model training device provided by an embodiment of the present invention, and the device is configured in a server. A federated model training device provided in an embodiment of the present invention can execute a federated model training method provided in any embodiment of the present invention, and the device includes:
[0099] The sending module 510 is configured to send the global model corresponding to the target task to each edge working node, and is also used to send the updated global model to each edge working node for the next round of local model training;
[0100] A determination module 520, configured to determine the specified number of local models participating in the global model update according to the current network resources and the number of target tasks based on a preset algorithm;
[0101] The current network resources include: current network bandwidth and current computing resourc...
Embodiment 3
[0120] Image 6 It is a schematic structural diagram of an electronic device provided by Embodiment 3 of the present invention. Image 6 A block diagram of an exemplary electronic device 12 suitable for use in implementing embodiments of the invention is shown. Image 6 The electronic device 12 shown is only an example, and should not limit the functions and scope of use of the embodiments of the present invention.
[0121] Such as Image 6 As shown, electronic device 12 takes the form of a general-purpose computing device. Components of electronic device 12 may include, but are not limited to, one or more processors or processing units 16, system memory 28, bus 18 connecting various system components including system memory 28 and processing unit 16.
[0122] Bus 18 represents one or more of several types of bus structures, including a memory bus or memory controller, a peripheral bus, an accelerated graphics port, a processor, or a local bus using any of a variety of bus ...
PUM
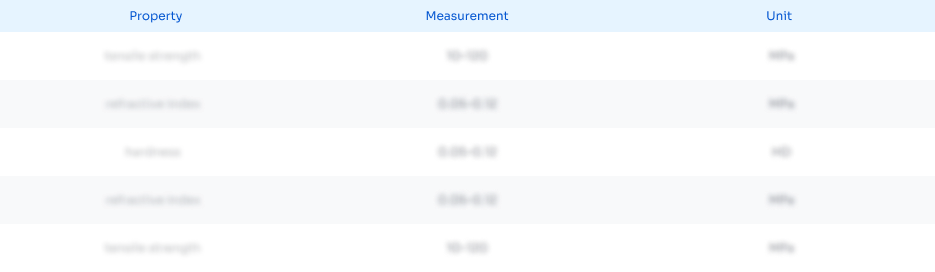
Abstract
Description
Claims
Application Information
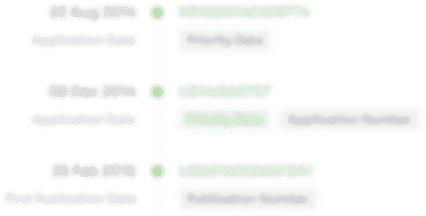
- R&D Engineer
- R&D Manager
- IP Professional
- Industry Leading Data Capabilities
- Powerful AI technology
- Patent DNA Extraction
Browse by: Latest US Patents, China's latest patents, Technical Efficacy Thesaurus, Application Domain, Technology Topic, Popular Technical Reports.
© 2024 PatSnap. All rights reserved.Legal|Privacy policy|Modern Slavery Act Transparency Statement|Sitemap|About US| Contact US: help@patsnap.com