An adversarial double-contrast self-supervised learning method for cross-modal lip reading
A supervised learning and adversarial technology, applied in the field of image processing, can solve problems such as dependence, neglect, and the validity of negative samples, and achieve the effect of optimizing representation learning
- Summary
- Abstract
- Description
- Claims
- Application Information
AI Technical Summary
Problems solved by technology
Method used
Image
Examples
Embodiment Construction
[0041] Such as figure 1 As shown, given a video of the mouth talking and the corresponding audio , first introduces a visual encoder and an audio encoder to extract the A-V embedding. To ensure consistent A-V embedding, both the audio encoder network and the visual encoder network ingest clips with the same duration, typically 0.2 seconds. Specifically, the input to the audio encoder is 13-dimensional Mel-frequency cepstral coefficients (MFCCs), which are extracted every 10ms with a frame length of 25ms. The input to the vision encoder is 5 consecutive mouth-centered cropped video (= 25) frames.
[0042] To learn effective visual representations for lip reading, three pre-tasks are introduced. Double Contrast Learning Objectives and The goal is to make the visual embeddings more closely resemble the corresponding audio embeddings on short and long time scales. adversarial learning objectives and Make the learned embedding independent of schema information a...
PUM
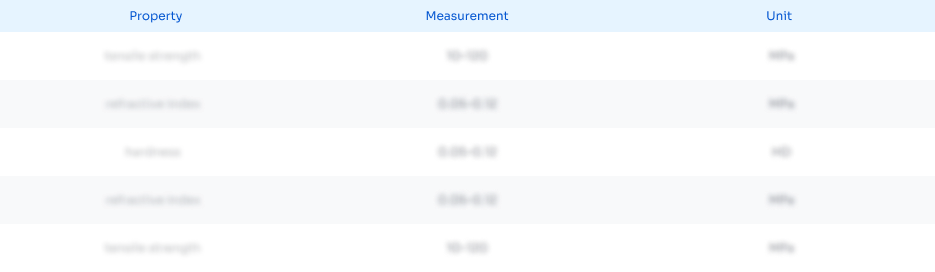
Abstract
Description
Claims
Application Information
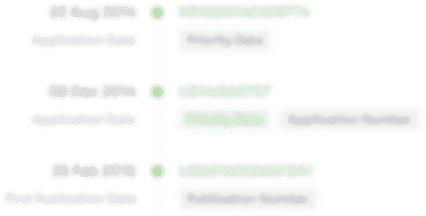
- R&D
- Intellectual Property
- Life Sciences
- Materials
- Tech Scout
- Unparalleled Data Quality
- Higher Quality Content
- 60% Fewer Hallucinations
Browse by: Latest US Patents, China's latest patents, Technical Efficacy Thesaurus, Application Domain, Technology Topic, Popular Technical Reports.
© 2025 PatSnap. All rights reserved.Legal|Privacy policy|Modern Slavery Act Transparency Statement|Sitemap|About US| Contact US: help@patsnap.com