Medical image super-resolution reconstruction method based on multi-attention residual feature fusion
A technology of super-resolution reconstruction and feature fusion, applied in neural learning methods, image data processing, graphics and image conversion, etc., can solve problems such as insufficient network representation ability and insufficient learning ability, so as to improve image super-resolution reconstruction performance, solve Effects of Insufficient Network Learning Ability
- Summary
- Abstract
- Description
- Claims
- Application Information
AI Technical Summary
Problems solved by technology
Method used
Image
Examples
Embodiment Construction
[0039] The present invention will be further described below in conjunction with the accompanying drawings.
[0040] refer to figure 1 with figure 2 , a medical image super-resolution reconstruction method based on multi-attention residual feature fusion, combining channel attention and spatial attention modules, weighting the features of the input image from multiple aspects, plus local and global residuals The features are fused and a novel super-resolution reconstruction method is constructed, including the following steps:
[0041] 1) Processing of training samples:
[0042] In order to make the proposed super-resolution network model have corresponding high-resolution images (i.e. HR) and low-resolution images (i.e. LR) in the retraining process, the present invention first performs a high-definition medical image original data set with a resolution of 512x512. 2x and 4x bicubic downsampling, resulting in low-resolution datasets at 256x256 and 128x128 resolutions.
...
PUM
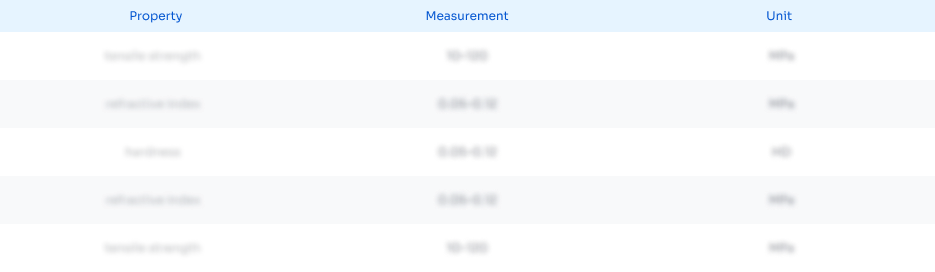
Abstract
Description
Claims
Application Information
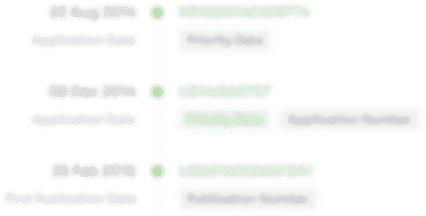
- Generate Ideas
- Intellectual Property
- Life Sciences
- Materials
- Tech Scout
- Unparalleled Data Quality
- Higher Quality Content
- 60% Fewer Hallucinations
Browse by: Latest US Patents, China's latest patents, Technical Efficacy Thesaurus, Application Domain, Technology Topic, Popular Technical Reports.
© 2025 PatSnap. All rights reserved.Legal|Privacy policy|Modern Slavery Act Transparency Statement|Sitemap|About US| Contact US: help@patsnap.com