Financial text entity relationship extraction method and system
An entity relationship and text technology, which is applied in the field of financial text entity relationship extraction method and system, can solve problems such as inflexibility, discarding, and inability to dynamically capture multi-hop relationships, and achieve the effect of enhancing modeling capabilities and improving performance
- Summary
- Abstract
- Description
- Claims
- Application Information
AI Technical Summary
Problems solved by technology
Method used
Image
Examples
Embodiment 1
[0076] Such as figure 1 As shown, a financial text entity relationship extraction method includes the following steps:
[0077] Step S1: Pre-train word embeddings using BERT on the financial dataset. The pre-training of BERT is divided into two stages, one is random masked word prediction, and the other is masked word prediction of financial entities;
[0078] Step S2: Establish an entity-relationship diagram. The entities in the financial text are regarded as nodes, and the texts where the entities are located are regarded as the relationship edges between the nodes. Entity nodes in the graph are coded in three categories: entities involved in relation extraction, start and end entities of text sequences related to entity relations, and irrelevant entities. The text where the entity is located is encoded by BERT stage 1 to obtain word embedding, and after the splicing position is embedded, it is sent to BiGRU encoding to obtain the relationship transition matrix between en...
Embodiment 2
[0082] Such as figure 2 As shown, a financial text entity relationship extraction system includes the following modules:
[0083] Word embedding pre-training module: From the financial text corpus, word embedding suitable for the financial field is trained, including the general word embedding of stage 1 and the financial entity word embedding of stage 2, for the construction of graph neural network;
[0084] Entity-relationship graph construction module: extract entities from financial texts as vertices, encode the text where the entities are located as edges, and construct entity-relationship graphs;
[0085] Entity-relationship diagram update module: disseminate information between entities, so that entity nodes in the entity-relationship diagram can obtain information about adjacent points;
[0086] Relationship prediction module: Embed entity pairs that need to be predicted from entity pairs and fusion representations between different layers, and predict the affiliatio...
PUM
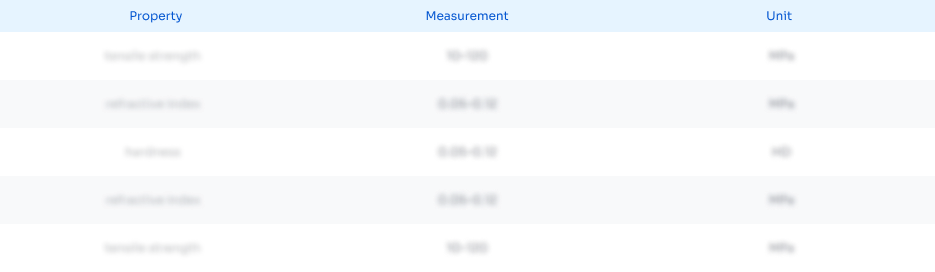
Abstract
Description
Claims
Application Information
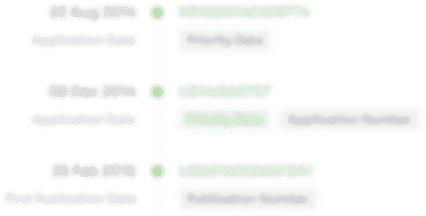
- R&D Engineer
- R&D Manager
- IP Professional
- Industry Leading Data Capabilities
- Powerful AI technology
- Patent DNA Extraction
Browse by: Latest US Patents, China's latest patents, Technical Efficacy Thesaurus, Application Domain, Technology Topic.
© 2024 PatSnap. All rights reserved.Legal|Privacy policy|Modern Slavery Act Transparency Statement|Sitemap