Graph neural network traffic flow prediction method based on multivariate time sequence interpolation
A multivariate time series and neural network technology, applied in the field of graph neural network traffic flow prediction based on multivariate time series interpolation, can solve problems such as data not real-time, traffic flow deviation, data loss, etc., and achieve the effect of overcoming data loss
- Summary
- Abstract
- Description
- Claims
- Application Information
AI Technical Summary
Problems solved by technology
Method used
Image
Examples
Embodiment Construction
[0042] In order to make the purpose, technical solution and advantages of the present invention clearer, the present invention will be further elaborated below in conjunction with the accompanying drawings.
[0043] In this example, see figure 1 with figure 2 As shown, the present invention proposes a graph neural network traffic flow prediction method based on multivariate time series interpolation, including steps:
[0044] S10, use a weighted graph to describe the topology between stations in the transportation network, and use the inflow and outflow of traffic as the characteristics of the station nodes themselves;
[0045] S20, constructing a traffic flow map according to the inflow and outflow of each station;
[0046] S30, using a graph neural network to interpolate missing values;
[0047] S40, obtaining a spatial feature sequence through a graph attention network;
[0048] S50, time attention captures the dynamic correlation between different times, and then uses...
PUM
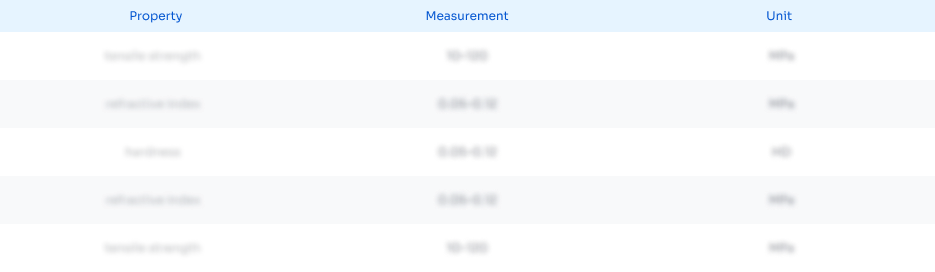
Abstract
Description
Claims
Application Information
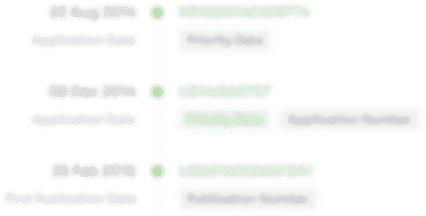
- R&D Engineer
- R&D Manager
- IP Professional
- Industry Leading Data Capabilities
- Powerful AI technology
- Patent DNA Extraction
Browse by: Latest US Patents, China's latest patents, Technical Efficacy Thesaurus, Application Domain, Technology Topic, Popular Technical Reports.
© 2024 PatSnap. All rights reserved.Legal|Privacy policy|Modern Slavery Act Transparency Statement|Sitemap|About US| Contact US: help@patsnap.com