Method for training multi-genus Boosting categorizer
A classifier and weak classifier technology, applied in the field of classifier training, can solve the problems of slow speed and low efficiency
- Summary
- Abstract
- Description
- Claims
- Application Information
AI Technical Summary
Problems solved by technology
Method used
Image
Examples
Embodiment Construction
[0030] The method for training a multi-class Boosting classifier of the present invention is based on the Boosting method, trains training data including training samples of multiple classes, and obtains weak classifiers for the plurality of classes to form a multi-class Boosting classification device. The method for training multi-class Boosting classifiers of the present invention is different from traditional Boosting methods in that before training, a performance target threshold is set, and when the strong classifier obtained after a certain training cycle is to the performance of a class When the performance target threshold is reached, the training for this class is completed, and the training samples of this class are removed from the training data. The remaining training samples are used in subsequent loops to train for the remaining classes. After each cycle in the training process and before the start of the next cycle, the sample weight of each training sample in ...
PUM
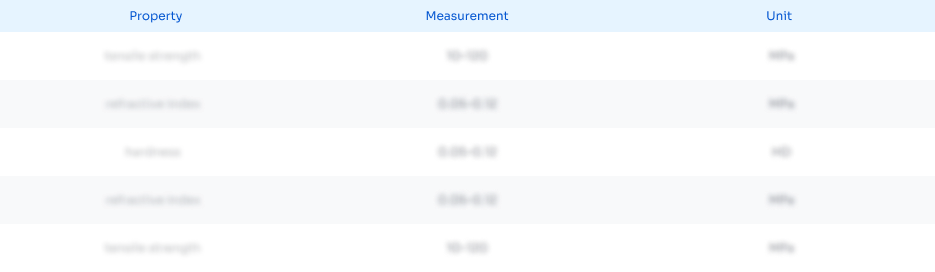
Abstract
Description
Claims
Application Information
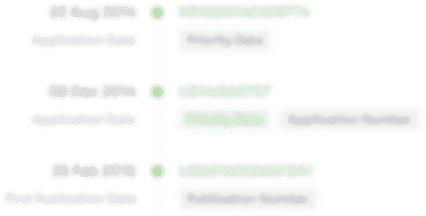
- R&D Engineer
- R&D Manager
- IP Professional
- Industry Leading Data Capabilities
- Powerful AI technology
- Patent DNA Extraction
Browse by: Latest US Patents, China's latest patents, Technical Efficacy Thesaurus, Application Domain, Technology Topic.
© 2024 PatSnap. All rights reserved.Legal|Privacy policy|Modern Slavery Act Transparency Statement|Sitemap