Feature parameter vector grouping quantization method in speech recognition
A speech recognition and feature parameter technology, applied in the field of biometrics, can solve problems such as the inability to ensure that the algorithm converges to the global optimum, and the large amount of calculation.
- Summary
- Abstract
- Description
- Claims
- Application Information
AI Technical Summary
Problems solved by technology
Method used
Image
Examples
specific Embodiment approach 1
[0011] Specific embodiment one: referring to Fig. 1, this embodiment consists of the following steps:
[0012] Step A1: Group all speech data participating in training according to their characteristics.
[0013] Step A2: Select the voice recognition information group to be processed, and set the required number N of codewords.
[0014] Step A3: extract the Mel frequency cepstral coefficients (MFCCs) of all speech signals contained in the current speech recognition information group.
[0015] Step A4: Randomly select the first C MFCCs of the current speech signal as the initial central values of the N MFCC codebooks, and the initial average distortion degree D old =∞; and set a cycle improvement parameter step, the initial value is 10 6 .
[0016] Step A5: according to the nearest neighbor rule based on the Euler distance, all the feature parameters MFCC of the current speech signal are mapped to the current V codeword regions to form N regions;
[0017] Step A6: Find ea...
specific Embodiment approach 2
[0025] Specific implementation mode two: this implementation mode further illustrates the specific method of the nearest neighbor method described in step A5 on the basis of specific implementation mode one:
[0026] Step B1: Calculate the distortion measure based on the Euclidean distance, the distortion measure is defined as the square of the distance between the vector X and the corresponding feature point in the feature space, d 2 ( x , z i ) = Σ k = 1 N ( x i - ( Z i ) K ) ...
specific Embodiment approach 3
[0028] Specific implementation mode three: this implementation mode further illustrates on the basis of specific implementation mode one that the specific method for eliminating the empty cell cavity and the average number of vectors in the cell cavity described in step A6 is:
[0029] Step C1: Find each region, if some region has no input vector into this category, it is determined that this region is an empty cavity, and marked.
[0030] Step C2: Find out the area with the largest number of vectors by item-by-item comparison, find out the feature vectors belonging to this area, divide the vector into this area, and classify it into the empty cell cavity marked in the previous step until the empty cell The number of vectors in the cavity is half of the number of vectors in the largest area.
[0031] Step C3: Find the center of the re-divided area to form a new codebook.
[0032] Step C4: Skip back to C1 until there is no empty cavity.
[0033] The above algorithm steps illu...
PUM
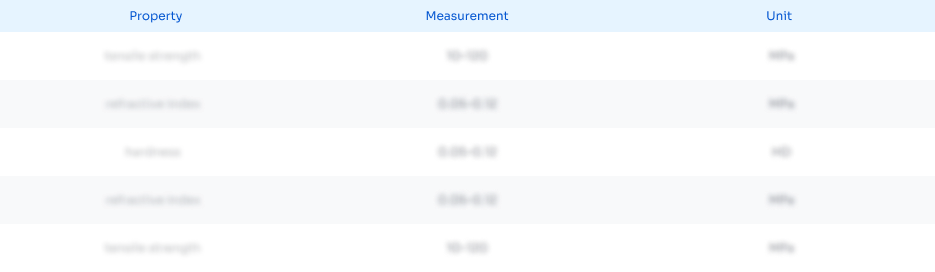
Abstract
Description
Claims
Application Information
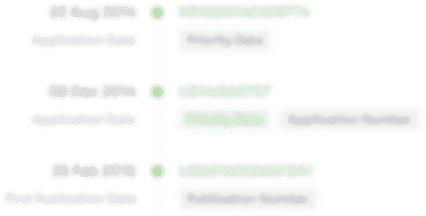
- R&D Engineer
- R&D Manager
- IP Professional
- Industry Leading Data Capabilities
- Powerful AI technology
- Patent DNA Extraction
Browse by: Latest US Patents, China's latest patents, Technical Efficacy Thesaurus, Application Domain, Technology Topic.
© 2024 PatSnap. All rights reserved.Legal|Privacy policy|Modern Slavery Act Transparency Statement|Sitemap