Image super-resolution rebuilding method based on dictionary learning and bilateral holomorphy
A technology of super-resolution reconstruction and dictionary learning, applied in the field of image processing, can solve the problems of reduced reconstruction effect and inability to guarantee reconstruction results, and achieve the effect of improving accuracy
- Summary
- Abstract
- Description
- Claims
- Application Information
AI Technical Summary
Problems solved by technology
Method used
Image
Examples
Embodiment Construction
[0025] Refer to attached figure 1 , the implementation steps of the present invention are as follows:
[0026] Step 1. For 5 interpolation images O 1 ,O 2 ,O 3 ,O 4 ,O 5 Perform adaptive clustering dictionary training to obtain the initial inner dictionary set d 0 and R cluster centers C center ={C i ,i=1,2,...,R}.
[0027] 1a) Extract 5 interpolation images respectively O 1 ,O 2 ,O 3 ,O 4 ,O 5 The high-frequency features of the corresponding high-frequency feature map G 1 ,G 2 ,G 3 ,G 4 ,G 5 ;
[0028] 1b) In the 5 interpolation maps O 1 ,O 2 ,O 3 ,O 4 ,O 5 and 5 high-frequency feature maps G 1 ,G 2 ,G 3 ,G 4 ,G 5 Take 7×7 image blocks; arrange all the image blocks acquired on these 5 interpolation maps in the form of column vectors to form a set of image blocks Arrange all the feature blocks obtained on these 5 high-frequency feature maps in the form of column vectors to form a set of feature blocks
[0029] 1c) Use K-means clustering method ...
PUM
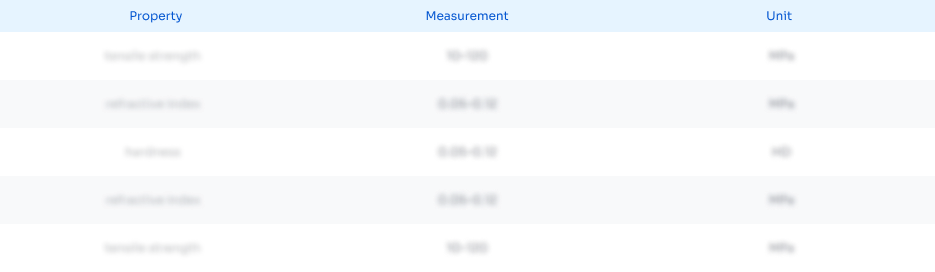
Abstract
Description
Claims
Application Information
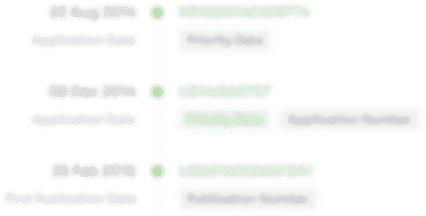
- R&D Engineer
- R&D Manager
- IP Professional
- Industry Leading Data Capabilities
- Powerful AI technology
- Patent DNA Extraction
Browse by: Latest US Patents, China's latest patents, Technical Efficacy Thesaurus, Application Domain, Technology Topic, Popular Technical Reports.
© 2024 PatSnap. All rights reserved.Legal|Privacy policy|Modern Slavery Act Transparency Statement|Sitemap|About US| Contact US: help@patsnap.com