Airport freight traffic prediction analysis method based on SARIMA and RBF neural network integration combination model
A neural network and combined model technology, applied in the field of transportation planning, design and management, can solve the problems that cannot meet the accuracy of the airport
- Summary
- Abstract
- Description
- Claims
- Application Information
AI Technical Summary
Problems solved by technology
Method used
Image
Examples
Embodiment Construction
[0045] After the airport has been in operation for many years, the airport cargo volume is affected by both linear factors such as fixed customer groups and the number of routes, as well as nonlinear factors such as the economy. The SARIMA-RBF model method provided by the present invention fully considers the influence of airport cargo volume The linear and nonlinear factors in order to improve the prediction accuracy of airport cargo traffic.
[0046] The experimental data of the present invention selects the real operating data of a domestic hub airport from January 2010 to July 2014, as shown in Table 1
[0047] Table 1 Cargo volume of a hub airport from January 2010 to July 2014
[0048]
[0049] In another embodiment of the present invention, normalization processing can also be performed on the time series data of airport cargo volume in advance.
[0050] Depend on figure 2 It can be clearly seen that the cargo volume of the airport has a clear upward trend from 20...
PUM
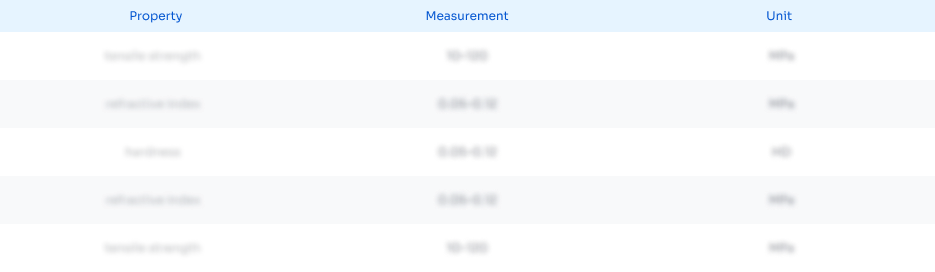
Abstract
Description
Claims
Application Information
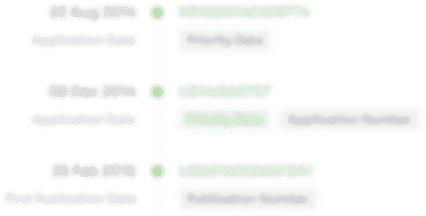
- R&D
- Intellectual Property
- Life Sciences
- Materials
- Tech Scout
- Unparalleled Data Quality
- Higher Quality Content
- 60% Fewer Hallucinations
Browse by: Latest US Patents, China's latest patents, Technical Efficacy Thesaurus, Application Domain, Technology Topic, Popular Technical Reports.
© 2025 PatSnap. All rights reserved.Legal|Privacy policy|Modern Slavery Act Transparency Statement|Sitemap|About US| Contact US: help@patsnap.com