Knowledge graph representation learning method
A knowledge map and vector technology, applied in the field of knowledge map representation learning, can solve the problem of not being able to accurately represent entities, relationships, and characteristics
- Summary
- Abstract
- Description
- Claims
- Application Information
AI Technical Summary
Problems solved by technology
Method used
Image
Examples
Embodiment 1
[0017] The invention discloses a knowledge graph representation learning method, and its flow diagram is as figure 2 As shown, the method includes:
[0018] Step 21: Use the translation-based model between the entity vector and the relationship vector to define the correlation between the entity vector and the relationship vector in the relationship triples (head, relationship, tail).
[0019] Among them, using the translation-based model between the entity vector and the relationship vector to define the correlation between the entity vector and the relationship vector in the relationship triplet includes:
[0020] S211. Define the probability of the relational triplet as p ( h | r , t , X ) = exp ( g ( h , r , t ) ) X h ‾ exp ( g ( h ‾ , r , t ) ) ;
[0021] Represent any entity in the knowledge graph; It is the normalization factor of the probability function of the relationship ...
Embodiment 2
[0045] Since the knowledge graph representation learning method of the present invention treats relationships and characteristics differently, further, the mutual connection between characteristics can be considered.
[0046] The knowledge graph representation learning method of the second embodiment of the present invention includes the following steps:
[0047] Step 31: Use the translation-based model between the entity vector and the relationship vector to define the correlation between the entity vector and the relationship vector in the relationship triples (head, relationship, tail).
[0048] Among them, using the translation-based model between the entity vector and the relationship vector to define the correlation between the entity vector and the relationship vector in the relationship triplet includes:
[0049] S311. Define the probability of the relational triplet as p ( h | r , t , X ) = exp ( g ( h , r , t ) ) X ...
PUM
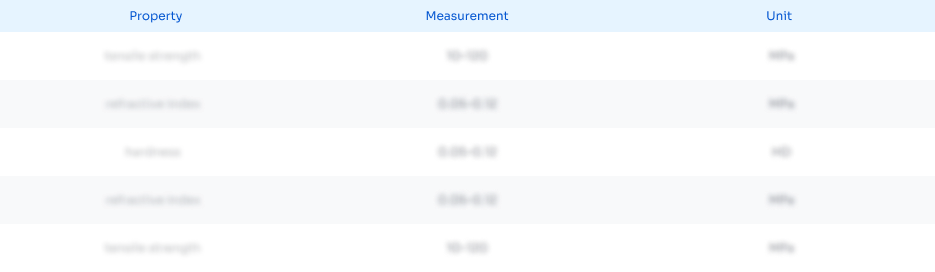
Abstract
Description
Claims
Application Information
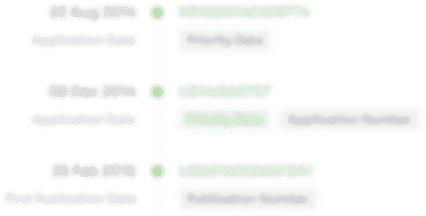
- R&D Engineer
- R&D Manager
- IP Professional
- Industry Leading Data Capabilities
- Powerful AI technology
- Patent DNA Extraction
Browse by: Latest US Patents, China's latest patents, Technical Efficacy Thesaurus, Application Domain, Technology Topic, Popular Technical Reports.
© 2024 PatSnap. All rights reserved.Legal|Privacy policy|Modern Slavery Act Transparency Statement|Sitemap|About US| Contact US: help@patsnap.com