A Polarized SAR Classification Method Based on Semi-Supervised Convolutional Neural Network
A technology of convolutional neural network and classification method, applied in the field of polarization SAR classification based on semi-supervised convolutional neural network, can solve the problems of large demand and low classification accuracy of label data
- Summary
- Abstract
- Description
- Claims
- Application Information
AI Technical Summary
Problems solved by technology
Method used
Image
Examples
Embodiment 1
[0113] Input the polarization SAR surface object simulation data to be classified, see image 3 (a), input the Pauli decomposition diagram of the polarimetric SAR and the coherence matrix T of the polarimetric SAR image, and obtain the label matrix Y according to the distribution information of the polarimetric SAR image, see image 3 (b), image 3 (b) is the image directly generated by the label matrix Y. Different color blocks in the image represent different ground features. The distribution of the same kind of ground features is represented by the same category label in the label matrix. The distribution of ground features that cannot be determined is in The label matrix is represented by 0, and the sample is generated by the coherence matrix T of the polarimetric SAR image combined with the Pauli decomposition diagram of the polarimetric SAR N is the total number of samples, x i represents the i-th sample.
[0114] Among them, the sample data are randomly extracted ...
Embodiment 2
[0128] 1. Experimental conditions
[0129] The hardware platform is: Intel(R) Core(TM) i5-2410M CPU@2.30GHz, RAM 4.00GB;
[0130] The software platform is: MATLAB R2016b;
[0131] The experiment selects 300×270 part of the polarized SAR ground object real data in the Flevoland area of the Netherlands for testing, and the number of categories is 6, namely Bare soil, Potato, Beet, Pea, Wheat and Barley. In the experiment, 1% of samples of each class are randomly selected as training samples, and the rest are testing samples.
[0132] 2. Experimental content and results
[0133] The present invention combines the Softmax classifier to classify the real data of polarized SAR surface objects, and compares it with other deep learning methods under the same experimental setting, wherein CNN is a convolutional neural network, Figure 4 (c) is determined by CNN Figure 4 (a) The result map of the classification; the experiment also uses the deep belief network WDBN based on Wisha...
PUM
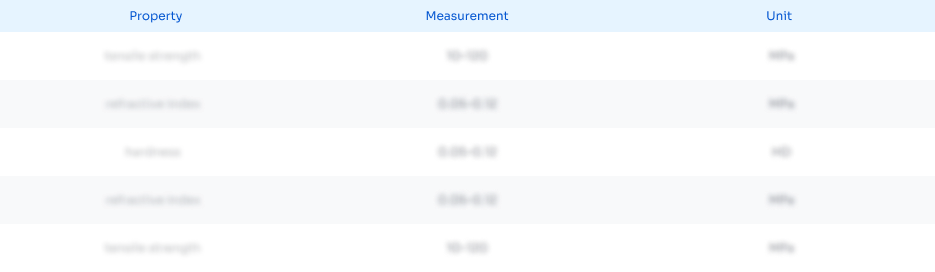
Abstract
Description
Claims
Application Information
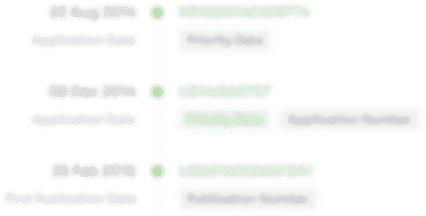
- R&D Engineer
- R&D Manager
- IP Professional
- Industry Leading Data Capabilities
- Powerful AI technology
- Patent DNA Extraction
Browse by: Latest US Patents, China's latest patents, Technical Efficacy Thesaurus, Application Domain, Technology Topic, Popular Technical Reports.
© 2024 PatSnap. All rights reserved.Legal|Privacy policy|Modern Slavery Act Transparency Statement|Sitemap|About US| Contact US: help@patsnap.com