Large-scale image multi-scale semantic retrieval method
A large-scale, picture technology, applied in still image data retrieval, metadata still image retrieval, character and pattern recognition, etc., can solve problems such as a lot of manpower, incomplete semantic representation, investment, etc.
- Summary
- Abstract
- Description
- Claims
- Application Information
AI Technical Summary
Problems solved by technology
Method used
Image
Examples
Embodiment Construction
[0027] The present invention will be further described below in conjunction with the drawings. As shown in the drawings, the specific implementation is divided into two parts: training and production environment. The training part is mainly to train the generative confrontation network. This training uses the tensorflow platform. The discriminator network is a convolutional neural network, and the generation network is a deconvolutional neural network. 64 images are used per iteration in the network. The main structure is attached figure 2 middle.
[0028] After the training is completed, get the trained model, and then use the trained model to build a standard tensorflow model server. In practical applications, one or a batch of pictures can be sent to the server each time to obtain the vector of the pictures.
[0029] After obtaining the picture vector, calculate the similarity with the picture to be searched, and find out the picture with a similarity greater than 0.5...
PUM
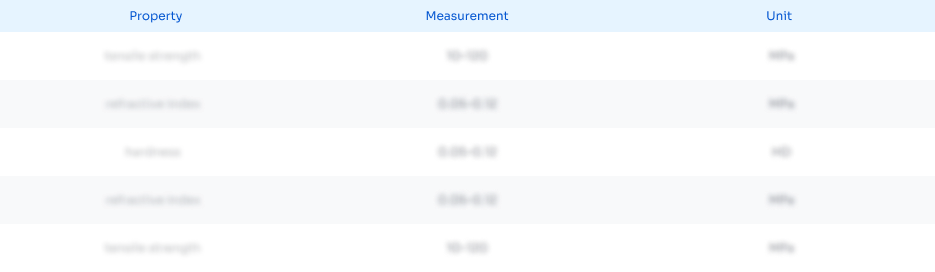
Abstract
Description
Claims
Application Information
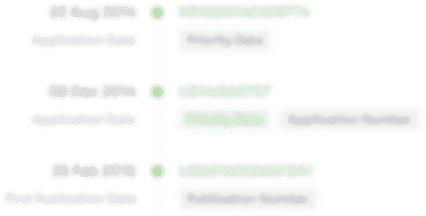
- R&D Engineer
- R&D Manager
- IP Professional
- Industry Leading Data Capabilities
- Powerful AI technology
- Patent DNA Extraction
Browse by: Latest US Patents, China's latest patents, Technical Efficacy Thesaurus, Application Domain, Technology Topic.
© 2024 PatSnap. All rights reserved.Legal|Privacy policy|Modern Slavery Act Transparency Statement|Sitemap