Human face identification method
A face recognition and face image technology, applied in the field of face recognition, can solve the problems of missing training sample set data, insufficient model performance, and low recognition accuracy, and achieve the effect of improving the face recognition rate
- Summary
- Abstract
- Description
- Claims
- Application Information
AI Technical Summary
Problems solved by technology
Method used
Image
Examples
Embodiment
[0041] The face recognition method of this embodiment, such as figure 1 shown, including the following steps:
[0042] The first step is to read the face image sample data set, each face image has 3 channels, its height is 112 pixels, and its width is 96 pixels.
[0043] In this embodiment, an open source face image sample data set is used, but the commonly used open source data set, whether it is the GG2 data set or the MS-Celeb-1M data set, has the problem of less data. In order to improve the generalization of this embodiment ability, it is necessary to merge the two data sets, but as mentioned in the background technology, if the two data sets are directly merged, the problem of sample overlap will be caused. Therefore, this embodiment adopts the following method for the GG2 data set It is still the MS-Celeb-1M data set for merging.
[0044] Specifically, as figure 2 As shown, in this embodiment, two face data sample sets VGG2 data set and MS-Celeb-1M data set are resp...
PUM
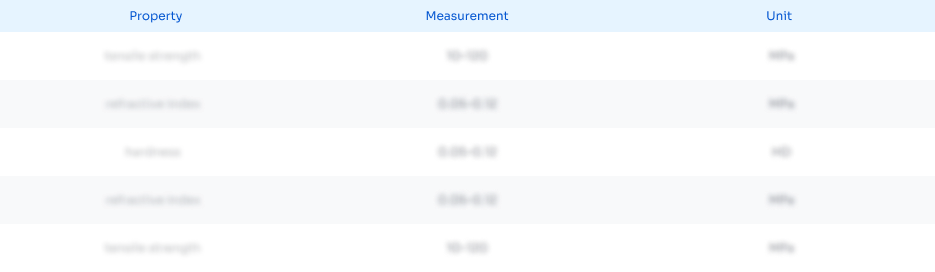
Abstract
Description
Claims
Application Information
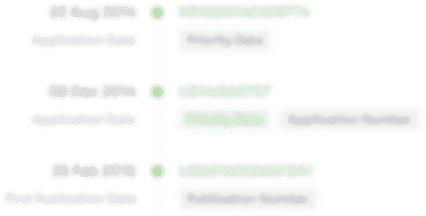
- R&D Engineer
- R&D Manager
- IP Professional
- Industry Leading Data Capabilities
- Powerful AI technology
- Patent DNA Extraction
Browse by: Latest US Patents, China's latest patents, Technical Efficacy Thesaurus, Application Domain, Technology Topic, Popular Technical Reports.
© 2024 PatSnap. All rights reserved.Legal|Privacy policy|Modern Slavery Act Transparency Statement|Sitemap|About US| Contact US: help@patsnap.com