Hyperspectral classification method based on dual branch network
A technology of hyperspectral classification and branch network, applied in the field of hyperspectral classification based on dual branch network, can solve problems such as poor classification ability, achieve the effects of maintaining diversity, improving classification accuracy, and alleviating the problem of sample imbalance
- Summary
- Abstract
- Description
- Claims
- Application Information
AI Technical Summary
Problems solved by technology
Method used
Image
Examples
Embodiment 1
[0082] The method of data resampling in the step (3) of the present invention, due to serious imbalance of given hyperspectral data, for example building and road have enough training samples, yet, water, unpaved parking lot and artificial turf are less than three thousand. Therefore, unbalanced samples will cause lower network performance. In order to solve this problem, the present invention proposes a resampling method, which specifically includes the following steps:
[0083] (3a) Randomly sample the same number of samples from each type of data and put them into a temporary data pool;
[0084] (3b) Randomly select a part of the data in the temporary data pool as the input of the network.
[0085] Through the method of resampling, it can ensure that the number of each category of input data in each iteration of the training process is not constant and equal, and it can also ensure that the samples of each category participating in the training are statistically balanced, ...
Embodiment 2
[0087] The method for extracting multi-scale features of data in the step (4) of the present invention is shown in the schematic diagram of the double-branch network structure. Through analysis, it has been shown that multi-scale features have played an important role in the solution of this problem. Therefore, the present invention has designed a double-branch network structure. , the input size of the entire network is a 17×17 image block, and its label is determined by the category of the center point of the image block. The network is mainly composed of two parts, including the following parts:
[0088] (4a) In the upper branch network, the image block is further cut to 16×16, and the convolution layer, normalization layer, activation function layer and pooling layer are processed, and the final feature is flattened to form a feature vector F1;
[0089] (4b) Compared with the upper branch network, the lower branch network pays more attention to multi-scale information. The...
PUM
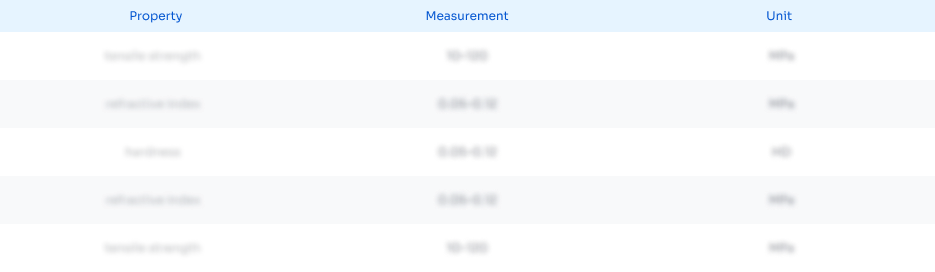
Abstract
Description
Claims
Application Information
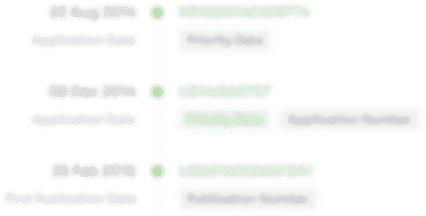
- Generate Ideas
- Intellectual Property
- Life Sciences
- Materials
- Tech Scout
- Unparalleled Data Quality
- Higher Quality Content
- 60% Fewer Hallucinations
Browse by: Latest US Patents, China's latest patents, Technical Efficacy Thesaurus, Application Domain, Technology Topic, Popular Technical Reports.
© 2025 PatSnap. All rights reserved.Legal|Privacy policy|Modern Slavery Act Transparency Statement|Sitemap|About US| Contact US: help@patsnap.com