A small sample depth learning method based on knowledge transfer of shallow model
A deep learning, small sample technology, applied in character and pattern recognition, instruments, computer parts, etc., can solve the problem that it is difficult to use and learn high-value sample information and features, lack of expert knowledge embedding and guidance, and feature representation distinguishability It is not strong enough to achieve the effect of good model representation ability and generalization ability, overcoming poor generalization ability, and overcoming insufficient model representation ability.
- Summary
- Abstract
- Description
- Claims
- Application Information
AI Technical Summary
Problems solved by technology
Method used
Image
Examples
Embodiment Construction
[0038] The present invention will be further described below in conjunction with accompanying drawing.
[0039] Such as figure 1 As shown, the present invention proposes a small-sample deep learning method based on shallow model knowledge transfer, including the following steps:
[0040] Step (1) data preprocessing
[0041] Building rotating machinery fault diagnosis datasets on a multifunctional motor platform, such as figure 2 As shown, 8 sensors are installed on the base to record vibration signals. In the experiment, 6 kinds of faults are considered: rotor unbalance 1 (RU1), rotor unbalance 3 (RU3), rotor unbalance 5 (RU5), rotor unbalance 7 (RU7), fan page break (PPB), loose base (PL). Record the data of each sample lasting 8s, which is 10240 data points. 300 samples were collected for each fault and normal condition. image 3 It shows the first sensor waveform of the above 6 kinds of rotating machinery faults and normal conditions.
[0042] For the original one-d...
PUM
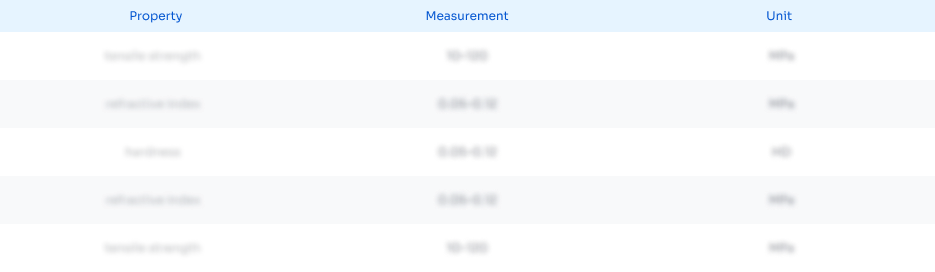
Abstract
Description
Claims
Application Information
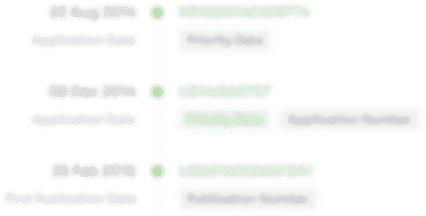
- Generate Ideas
- Intellectual Property
- Life Sciences
- Materials
- Tech Scout
- Unparalleled Data Quality
- Higher Quality Content
- 60% Fewer Hallucinations
Browse by: Latest US Patents, China's latest patents, Technical Efficacy Thesaurus, Application Domain, Technology Topic, Popular Technical Reports.
© 2025 PatSnap. All rights reserved.Legal|Privacy policy|Modern Slavery Act Transparency Statement|Sitemap|About US| Contact US: help@patsnap.com