Artificial neural network with side input for language modelling and prediction
An artificial neural network, neural network technology, applied in the field of artificial neural network with secondary input for language modeling and prediction, can solve problems such as dependence on statistical correlation
- Summary
- Abstract
- Description
- Claims
- Application Information
AI Technical Summary
Problems solved by technology
Method used
Image
Examples
Embodiment Construction
[0040] figure 1 A simple artificial neural network 100 depicts the state of the prior art. In essence, artificial neural network such as artificial neural network 100 is a mathematical functional chain tissue in the direction dependent layer, said direction dependent layer, such as input layer 101, hiding layer 102, and output layer 103, each layer Includes multiple units or nodes, 110-131. Artificial neural network 100 is called a "feedforward neural network", which is because the output of each layer 101-103 is used as input (or, in the case of output layer 103, it is artificial neural network 100). Output) and there is no backward step or loop. It should be understood that figure 1 The number of units 110-131 depicted in the present is exemplary, and the general artificial neural network includes more units in each layer 101-103.
[0041] In the operation of artificial neural network 100, an input is provided at the output layer 101. This generally involves mapping the real wo...
PUM
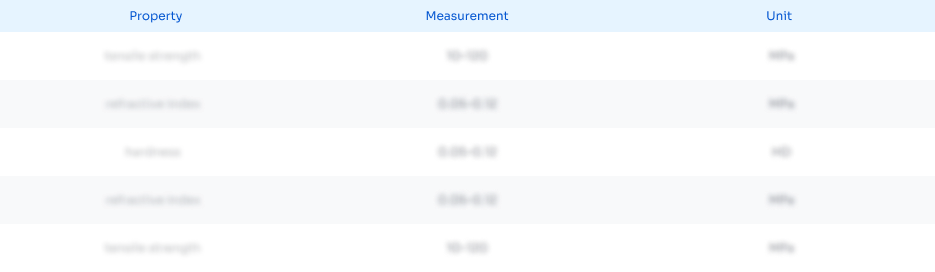
Abstract
Description
Claims
Application Information
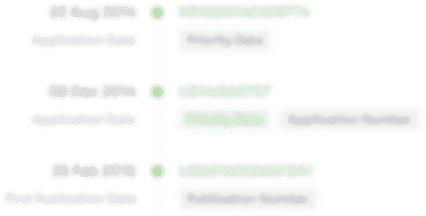
- R&D
- Intellectual Property
- Life Sciences
- Materials
- Tech Scout
- Unparalleled Data Quality
- Higher Quality Content
- 60% Fewer Hallucinations
Browse by: Latest US Patents, China's latest patents, Technical Efficacy Thesaurus, Application Domain, Technology Topic, Popular Technical Reports.
© 2025 PatSnap. All rights reserved.Legal|Privacy policy|Modern Slavery Act Transparency Statement|Sitemap|About US| Contact US: help@patsnap.com