A Zero-Shot Image Classification Method Based on Adversarial Autoencoder Model
A self-encoder and sample image technology, applied to biological neural network models, instruments, computer components, etc., can solve the problems of lack of discriminative information for visual features, ignoring the correspondence between visual features and category semantic features, etc.
- Summary
- Abstract
- Description
- Claims
- Application Information
AI Technical Summary
Problems solved by technology
Method used
Image
Examples
Embodiment Construction
[0032] A zero-shot image classification method based on the adversarial autoencoder model of the present invention will be described in detail below with reference to the embodiments and the accompanying drawings.
[0033] A zero-shot image classification method based on the adversarial autoencoder model of the present invention assumes that the reverse process of generating category semantic features from visual features is considered while using category semantic features to generate visual features. Therefore, on the basis of using the confrontational network, the self-encoder is introduced to complete the two-way generation process through its encoding and decoding process, so as to achieve the purpose of generating visual features and associating visual features with category semantic features.
[0034]An autoencoder is a type of neural network that is trained to copy an input to an output. The self-encoder consists of two parts, the encoder h=E(x) and the decoder x'=G(h)...
PUM
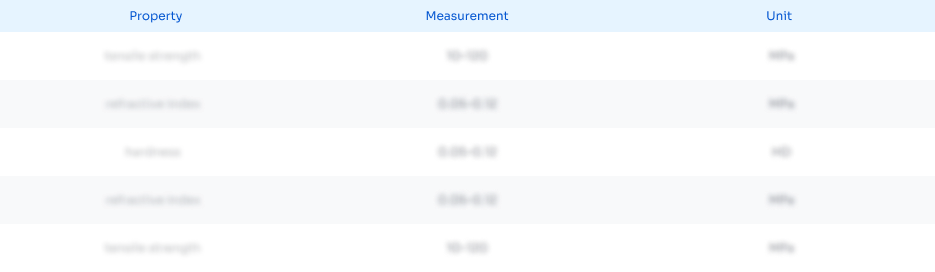
Abstract
Description
Claims
Application Information
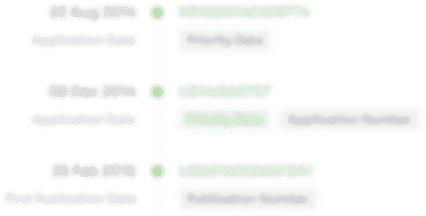
- R&D
- Intellectual Property
- Life Sciences
- Materials
- Tech Scout
- Unparalleled Data Quality
- Higher Quality Content
- 60% Fewer Hallucinations
Browse by: Latest US Patents, China's latest patents, Technical Efficacy Thesaurus, Application Domain, Technology Topic, Popular Technical Reports.
© 2025 PatSnap. All rights reserved.Legal|Privacy policy|Modern Slavery Act Transparency Statement|Sitemap|About US| Contact US: help@patsnap.com