Dynamic classifier chain adjusting method for multi-label classification
A dynamic classification and adjustment method technology, applied in the direction of instruments, character and pattern recognition, computer components, etc., to achieve the effect of alleviating randomness
- Summary
- Abstract
- Description
- Claims
- Application Information
AI Technical Summary
Problems solved by technology
Method used
Image
Examples
Embodiment 1
[0027] The dynamic classifier chain adjustment method for multi-label classification of the present invention, the method is in the training data set, respectively counts the number of times each marker and other markers co-occur and sorts them in descending order, and completes the classifier in the training data set Chain sorting, and then randomly select a classifier from the classifier chain to complete the classification of unknown samples. When classifying unknown samples, set two thresholds in advance, according to the output value of the randomly selected classifier and the two thresholds Complete the classification of unknown samples, reduce the probability of misclassification of unknown samples whose output is near the threshold when classifying unknown samples, and alleviate the randomness of the label prediction sequence and the uncertainty of classification results in the classifier chain sex and instability.
Embodiment 2
[0029] The ordering of classifier chains of the present invention refers to: each classifier is a secondary classifier and corresponds to each category, the number of classifier chains is equal to the number of categories, and the first classifier of each classifier chain corresponds to the first classifier. categories.
[0030] The sorting method of the classifier chain includes the following steps:
[0031] (1), mark a category in the training data set as l i ;
[0032] (2), from all have mark l i In the samples, the statistics have the exception mark l i The number of samples marked other than ;
[0033] (3), in descending order, mark l i To sort by tags other than the tag l i Arranged in the first position of the classifier chain, the order of the subsequent classifiers can be sorted according to the marks.
[0034] More preferably, the relationship between the classifier chain and the classifier is specifically as follows: there are n category marks {1 1 , l 2 .....
Embodiment 3
[0036] The classification method of the unknown sample of the present invention specifically includes the following steps:
[0037] (i) When classifying unknown samples, randomly select a classifier C i ;
[0038] (ii), according to C i The output of f(C i ) value and threshold a and threshold b to select the classification of unknown samples:
[0039] ①, when C i When the output value is greater than the threshold b, then according to C i The corresponding classifier chain R(C i ), to classify unknown samples;
[0040] ②, when C i When the output value is less than the threshold a, then from C i The corresponding classifier chain R(C i ) at the end to select the corresponding secondary classifier classification in turn, until a classifier C with output greater than the threshold appears j , then follow C j The corresponding classifier chain R(C j ), to classify unknown samples;
[0041] ③, when C j When the output value of is between a and b, reselect another cl...
PUM
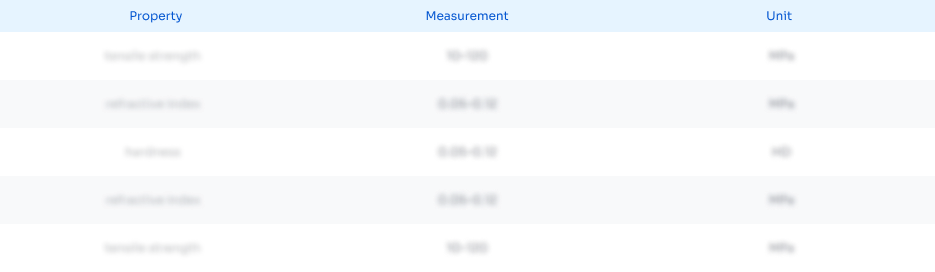
Abstract
Description
Claims
Application Information
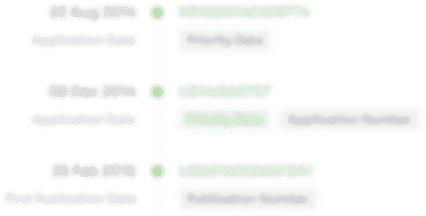
- R&D
- Intellectual Property
- Life Sciences
- Materials
- Tech Scout
- Unparalleled Data Quality
- Higher Quality Content
- 60% Fewer Hallucinations
Browse by: Latest US Patents, China's latest patents, Technical Efficacy Thesaurus, Application Domain, Technology Topic, Popular Technical Reports.
© 2025 PatSnap. All rights reserved.Legal|Privacy policy|Modern Slavery Act Transparency Statement|Sitemap|About US| Contact US: help@patsnap.com