Multi-tag entity-relationship joint extraction method based on depth neural network and annotation strategy
A deep neural network and joint extraction technology, which is applied in the field of multi-label entity-relationship joint extraction based on deep neural network and labeling strategy, can solve problems such as failure to solve relationship overlap well, failure to achieve joint extraction, etc.
- Summary
- Abstract
- Description
- Claims
- Application Information
AI Technical Summary
Problems solved by technology
Method used
Image
Examples
Embodiment
[0044] Embodiment: It is mainly used to extract the entities in each sentence of the data set and the semantic relationship of the entities. Both training data and test data are selected from the NYT dataset.
[0045] Such as figure 1 The process shown and figure 2 As shown in the model, the method of the present invention comprises the following steps,
[0046] Step 1: Firstly, word segmentation is performed on the training text and test text, and the training text obtained after word segmentation is marked with a marking strategy. The marking strategy is specifically: according to the labeling of the training text, set an "O" label (not belonging to any relationship) or a "non-O" label (having a relationship) for each word according to the labeling of the training text. The non-O label consists of three parts: word position, relation category and relation role. Wherein, the word position marks include B (begin), I (inside), E (end) and S (single), which are used to repr...
PUM
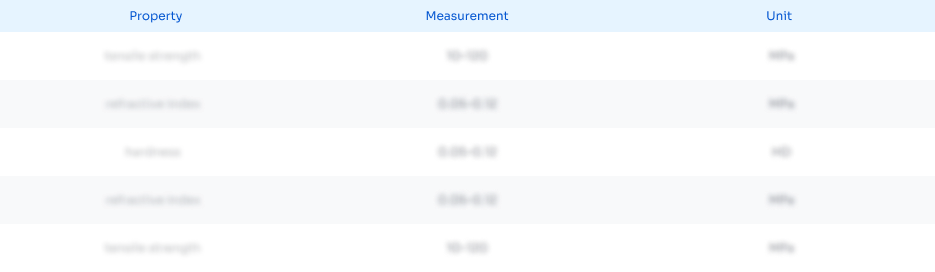
Abstract
Description
Claims
Application Information
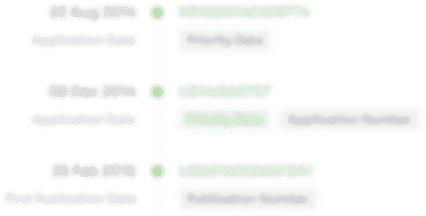
- R&D Engineer
- R&D Manager
- IP Professional
- Industry Leading Data Capabilities
- Powerful AI technology
- Patent DNA Extraction
Browse by: Latest US Patents, China's latest patents, Technical Efficacy Thesaurus, Application Domain, Technology Topic, Popular Technical Reports.
© 2024 PatSnap. All rights reserved.Legal|Privacy policy|Modern Slavery Act Transparency Statement|Sitemap|About US| Contact US: help@patsnap.com