A domain adaptive deep learning method and a readable storage medium
A deep learning and self-adaptive technology, applied in neural learning methods, instruments, biological neural network models, etc., can solve problems such as the model is not optimal, convergence is more difficult than non-confrontational training, and achieve the effect of improving performance
- Summary
- Abstract
- Description
- Claims
- Application Information
AI Technical Summary
Problems solved by technology
Method used
Image
Examples
Embodiment Construction
[0020] The present invention will be described in further detail below in conjunction with the accompanying drawings.
[0021] figure 1 A schematic diagram of the domain-adaptive deep learning training process of the embodiment of the present invention is given. The method mainly includes the following steps:
[0022] Step 1: Rotate and transform the target domain image to obtain a self-supervised learning training sample set;
[0023] Step 2: Jointly train the converted self-supervised learning training sample set and source domain training samples to obtain a deep learning model.
[0024] Step 3: Use the model obtained from the above joint training for the vision task T on the target domain.
[0025] In step 1, the target domain image is rotated and transformed to obtain a training sample set for self-supervised learning. The process first rotates the target domain image by 0°, 90° and 180° respectively, and the three rotation angles correspond to category labels 0, 1 an...
PUM
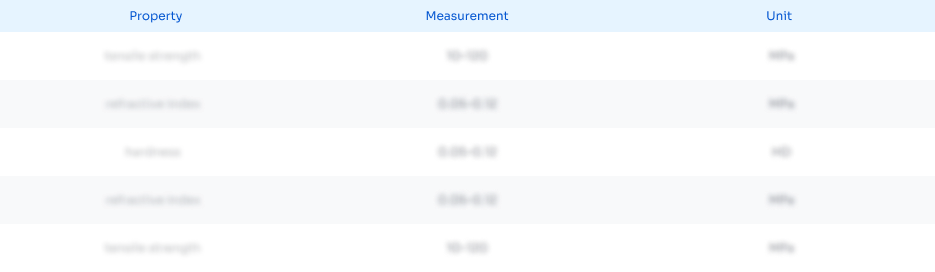
Abstract
Description
Claims
Application Information
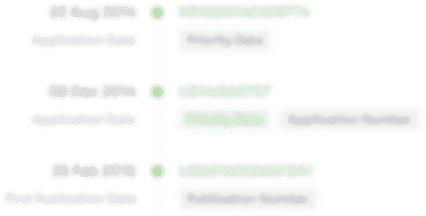
- R&D Engineer
- R&D Manager
- IP Professional
- Industry Leading Data Capabilities
- Powerful AI technology
- Patent DNA Extraction
Browse by: Latest US Patents, China's latest patents, Technical Efficacy Thesaurus, Application Domain, Technology Topic, Popular Technical Reports.
© 2024 PatSnap. All rights reserved.Legal|Privacy policy|Modern Slavery Act Transparency Statement|Sitemap|About US| Contact US: help@patsnap.com