A Self-Supervised Domain Adaptive Deep Learning Method Based on Consistency Training
A deep learning and consistency technology, applied in neural learning methods, biological neural network models, instruments, etc., can solve problems such as difficulty in obtaining models and difficulty in convergence
- Summary
- Abstract
- Description
- Claims
- Application Information
AI Technical Summary
Problems solved by technology
Method used
Image
Examples
Embodiment Construction
[0033] The present invention will be further described in detail below in conjunction with the attached drawings and examples of self-adaptive learning in the field of image classification, but this does not limit the protection scope of the present invention.
[0034] figure 1 A schematic diagram of the self-supervised domain adaptive deep learning training process based on consistency training in the embodiment of the present invention is given. Adaptive learning in the field of image classification mainly includes the following steps:
[0035] S1: Construct a multi-task learning deep neural network, including a parameter θ e The feature extraction network E of the parameter is θ m Image classification network M, and the parameter is θ p The image enhancement transform prediction network P;
[0036] The image enhancement transformation in S1 in this embodiment adopts an image rotation operation.
[0037] S2: Transform the source domain image x s and its category label ...
PUM
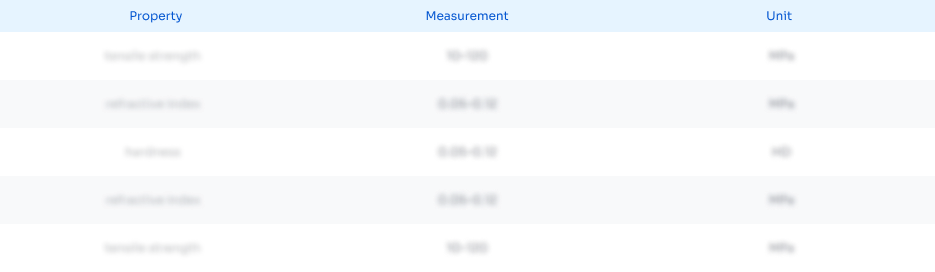
Abstract
Description
Claims
Application Information
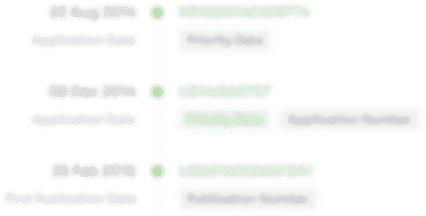
- R&D Engineer
- R&D Manager
- IP Professional
- Industry Leading Data Capabilities
- Powerful AI technology
- Patent DNA Extraction
Browse by: Latest US Patents, China's latest patents, Technical Efficacy Thesaurus, Application Domain, Technology Topic, Popular Technical Reports.
© 2024 PatSnap. All rights reserved.Legal|Privacy policy|Modern Slavery Act Transparency Statement|Sitemap|About US| Contact US: help@patsnap.com