Video action migration method
A video, action technology, applied in the field of computer vision, can solve problems such as lack of effective methods
- Summary
- Abstract
- Description
- Claims
- Application Information
AI Technical Summary
Problems solved by technology
Method used
Image
Examples
Embodiment 1
[0029] The problem addressed by this application is located in the migration of human actions in videos. V={I 1 , I 2 ,...,I N} represents an N-frame video in which a single person performs whole-body movements, such as dancing. To simplify the problem, it is assumed that both the viewpoint (camera) and the background are stationary, even so, it remains an unsolved challenging problem. Given a source video V S and the target action video V T , the goal of action transfer is to put V T The action migrates to V S , while maintaining V S appearance characteristics. In this way, for the generated target video V O , you can control both motion and appearance while displaying. A pre-trained 2D pose detection model is used to extract the action sequences P={p 1 ,p 2 ,...,p N}. each p tIndicates the posture of the t-th frame, and the representation in the implementation is a thermal value map of M channels, where M=14 represents the number of key points. Denote the sou...
Embodiment 2
[0071] This application uses PSNR and VFID as evaluation indicators. To calculate the VFID, first use a pre-trained video classification model I3D to extract video features, and then calculate the mean and covariance matrix on all videos in the dataset The final VFID is calculated by the formula:
[0072]
[0073] VFID measures both visual effect and temporal continuity.
[0074]For transfer within the same video, the real video is the target video, and PSNR and VFID can be easily calculated. For cross-video transfer, PSNR cannot be calculated because there is no real frame correspondence. At the same time, the reference significance of VFID is also greatly reduced, because the appearance and background will also greatly affect the features extracted by the I3D network. Therefore, only quantitative results of in-video action transfer are provided.
[0075] Table 1 Quantitative results
[0076]
[0077] The above table shows the PSNR and VFID scores of different met...
Embodiment 3
[0087] This application has also done a qualitative experiment. Two scenarios of intra-video action migration and cross-video action migration were tested respectively. These two scenarios correspond to two different test subsets: i) Cross-video test set, source character / background frame and target action video are from different video sequences. ii) In-video test set, source person / background frames and target action videos are from the same video sequence. For each set, 50 pairs of videos were randomly selected from the test set as a test subset. Note that in the intra-video test subset, it is ensured that the source and target sequences do not intersect or overlap.
[0088] In the results generated by the base model for a single frame, obvious blurring and unnaturalness can be observed.
[0089] The results of the max pooling fusion method tend to generate strange colors and shadows in the foreground and background, and the reason is guessed to be the persistence effect...
PUM
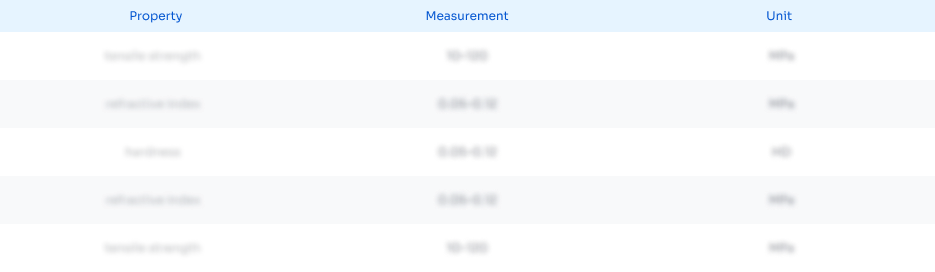
Abstract
Description
Claims
Application Information
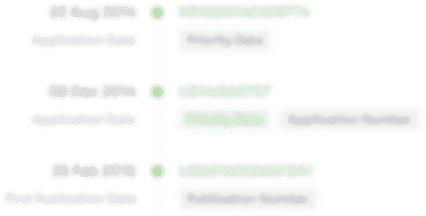
- R&D Engineer
- R&D Manager
- IP Professional
- Industry Leading Data Capabilities
- Powerful AI technology
- Patent DNA Extraction
Browse by: Latest US Patents, China's latest patents, Technical Efficacy Thesaurus, Application Domain, Technology Topic.
© 2024 PatSnap. All rights reserved.Legal|Privacy policy|Modern Slavery Act Transparency Statement|Sitemap