Named entity identification method based on time convolution network
A named entity recognition and convolutional network technology, applied in neural learning methods, biological neural network models, special data processing applications, etc., can solve problems such as slow running speed, achieve increased size, improved flexibility, training and verification The effect of shortening time
- Summary
- Abstract
- Description
- Claims
- Application Information
AI Technical Summary
Benefits of technology
Problems solved by technology
Method used
Image
Examples
Embodiment
[0033] Example: see figure 1 , Is the overall architecture of the model proposed by the present invention, which is mainly composed of three parts: the feature presentation layer, the TCN and the CRF layer. Among them, the feature representation layer is mainly composed of word vectors and character feature layers. The word vector layer and the character vector layer accept words and characters as input respectively, and map the discrete One-hot representations to their respective continuous dense low-dimensional feature spaces; then, the word vectors and character-level vectors are spliced to represent words Features in a specific semantic space; then the spliced features are used as the input of TCN, and different features extracted by TCNs with different convolution kernel sizes are merged to obtain the final feature h 1 h 2 ...h n , And use this as the input of the CRF layer. After CRF further restricts the context labeling, the output sequence labeling result y 1 y 2 ....
PUM
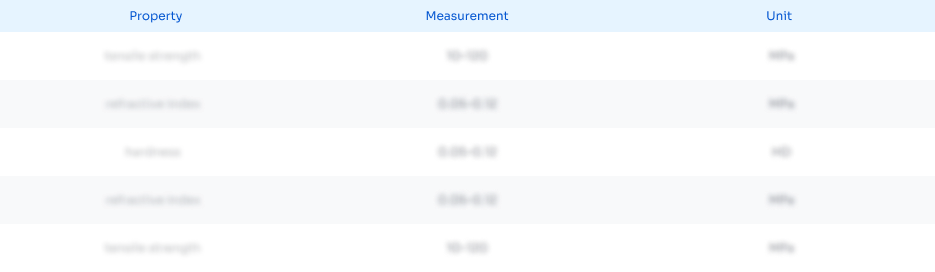
Abstract
Description
Claims
Application Information
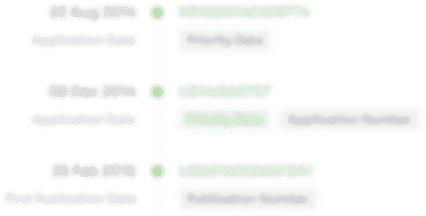
- R&D
- Intellectual Property
- Life Sciences
- Materials
- Tech Scout
- Unparalleled Data Quality
- Higher Quality Content
- 60% Fewer Hallucinations
Browse by: Latest US Patents, China's latest patents, Technical Efficacy Thesaurus, Application Domain, Technology Topic, Popular Technical Reports.
© 2025 PatSnap. All rights reserved.Legal|Privacy policy|Modern Slavery Act Transparency Statement|Sitemap|About US| Contact US: help@patsnap.com