A Face Age Estimation Method Based on Self-paced Learning
A face and normalization technology, applied in the fields of social network entertainment and face age estimation, can solve the problems of poor prediction effect of small data sets, high hardware configuration requirements, etc., to improve learning robustness and powerful representation ability Effect
- Summary
- Abstract
- Description
- Claims
- Application Information
AI Technical Summary
Problems solved by technology
Method used
Image
Examples
Embodiment Construction
[0122] The present invention is based on the face age estimation method of the depth regression forest of self-step learning, and its realization comprises the following steps:
[0123] Step 1: Preprocess the dataset;
[0124] For Moprh II ( http: / / www.faceaginggroup.com / morph / ) face database uses MTCNN to detect facial feature points, and obtains 5 facial feature points; according to the obtained 5 facial feature point positioning results, the image is normalized to a 224*224*3 RGB image; Processed 55,130 face images with age labels.
[0125] Step 2: Build a deep regression forest;
[0126] image 3 Represents the general structure of the deep regression forest, where the circle represents the feature value output by the last fully connected layer of the convolutional neural network, the square box represents the separation node of each tree, and the diamond box represents the leaf node of each tree;
[0127] The depth regression forest input is the eigenvalue of the la...
PUM
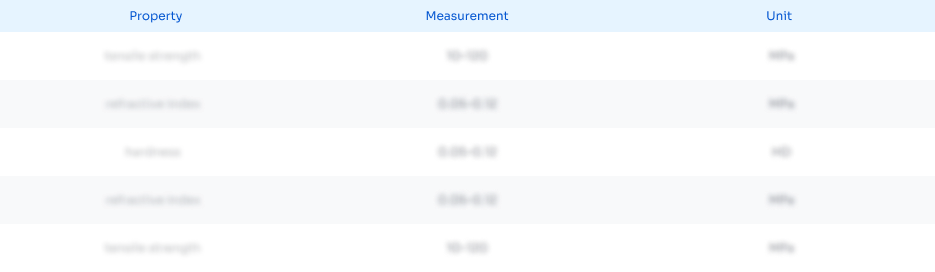
Abstract
Description
Claims
Application Information
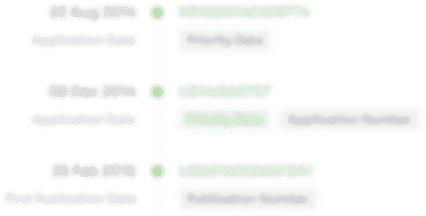
- R&D Engineer
- R&D Manager
- IP Professional
- Industry Leading Data Capabilities
- Powerful AI technology
- Patent DNA Extraction
Browse by: Latest US Patents, China's latest patents, Technical Efficacy Thesaurus, Application Domain, Technology Topic, Popular Technical Reports.
© 2024 PatSnap. All rights reserved.Legal|Privacy policy|Modern Slavery Act Transparency Statement|Sitemap|About US| Contact US: help@patsnap.com