A Weak Target Detection Method Based on Feature Mapping Neural Network
A weak target and neural network technology, applied in the field of weak target detection, can solve the problem of low detection accuracy, achieve high detection accuracy, improve network stability, and strong robustness
- Summary
- Abstract
- Description
- Claims
- Application Information
AI Technical Summary
Problems solved by technology
Method used
Image
Examples
Embodiment 1
[0096] The training data of the network is as image 3 As shown, the small circle represents one type of item, and the x point represents another type of sample. The training samples are linear and inseparable, which is difficult to distinguish; after training the deep network through the training samples, the second layer and the third layer are output Features such as Figure 4 As shown, after the linearly inseparable two-dimensional features are mapped to three-dimensional by the neural network, they have linear discriminability in the three-dimensional feature space, and after the three-dimensional features are re-encoded to two-dimensional, as Figure 5 As shown, the original non-linear and non-separable low-dimensional features are mapped to the linear discriminable feature space, and the blue x-point samples are distributed in a compact area. Such as figure 2 As shown, the entire network contains 6 layers in total, which can be divided into 2 parts. The input layer t...
Embodiment 2
[0106] Step 1 includes the following steps:
[0107] Step 1.1: Construct the structure of a spindle-type deep neural network including an input layer, a decoding layer, an encoding layer and a softmax output layer;
[0108] Step 1.2: using the cross-validation method to determine the hyperparameters of the spindle-shaped deep neural network to obtain the spindle-shaped deep neural network;
[0109] Step 1.3: Construct the training data set;
[0110] Step 1.4: Input the training data set into the spindle-type deep neural network and train in an unsupervised manner to obtain initialized network weights to complete the training.
[0111] The decoding layer and encoding layer training calculation are as follows:
[0112] h k =σ(W k X+b k )
[0113] Among them, W k represents the weight matrix, b k Represents the bias vector, σ represents the activation function, X={x 1 ,x 2 ,...,x m} represents the input of the current layer, h k Indicates the output of the current lay...
PUM
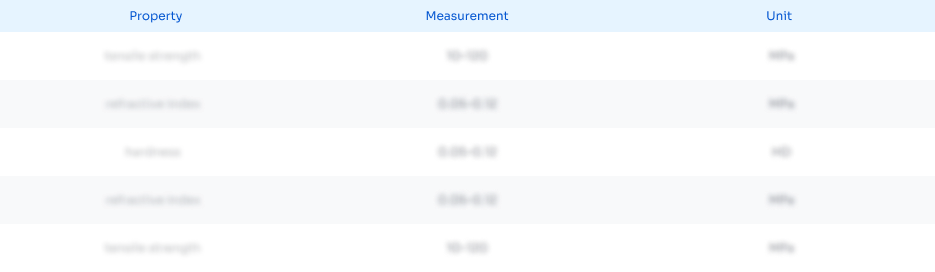
Abstract
Description
Claims
Application Information
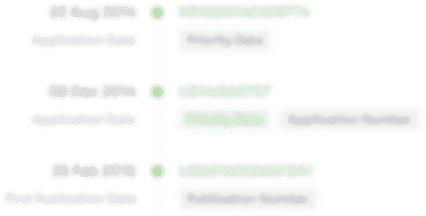
- R&D Engineer
- R&D Manager
- IP Professional
- Industry Leading Data Capabilities
- Powerful AI technology
- Patent DNA Extraction
Browse by: Latest US Patents, China's latest patents, Technical Efficacy Thesaurus, Application Domain, Technology Topic, Popular Technical Reports.
© 2024 PatSnap. All rights reserved.Legal|Privacy policy|Modern Slavery Act Transparency Statement|Sitemap|About US| Contact US: help@patsnap.com