Model training method and image change analysis method based on semi-supervised confrontation learning
A semi-supervised, image-based technology, applied in the field of remote sensing image processing and analysis and computer vision, can solve the problems of heavy workload, time-consuming, high accuracy and other problems of data sets, and achieve accurate and refined classification, reduce workload, and be easy to operate Effect
- Summary
- Abstract
- Description
- Claims
- Application Information
AI Technical Summary
Problems solved by technology
Method used
Image
Examples
Embodiment 1
[0066] Embodiments of the present invention provide a training method for a classification model of image feature elements based on semi-supervised confrontation learning, the basic flow of which is as follows figure 1 shown, including the following steps:
[0067] S11. Acquire a multi-temporal image to be trained; the multi-temporal image to be trained includes an annotated image and an unlabeled image for pixel-level annotation;
[0068] S12. Perform adversarial training on the annotated image and the first segmentation prediction map obtained based on the annotated image, the adversarial training includes a semantic segmentation network and a discriminant network;
[0069] S13. Input the unlabeled image into the discrimination network after adversarial training, obtain the trustworthy area in the unlabeled image close to the marked image, and use the trustworthy area as a supervisory signal to conduct semi-supervised training on the semantic segmentation network after adver...
Embodiment 2
[0139] An embodiment of the present invention provides an image change analysis method based on semi-supervised adversarial learning, the basic process of which is as follows Figure 6 shown, including:
[0140] S21. Obtain a multi-temporal image to be analyzed;
[0141] S22 Obtain the classification results of various ground features in the multi-temporal image through the trained image feature element classification model;
[0142] S23. According to the classification result, analyze the changes of various feature elements in the target area;
[0143] Wherein, the image feature element classification model is obtained through training using the method in Embodiment 1.
[0144] Since it is possible that the size of the original image is too large, the image can be preprocessed before forming a training set containing multi-temporal images to be trained. Therefore, before the above S21, it can also include:
[0145] Perform image enhancement on the multi-temporal images to ...
PUM
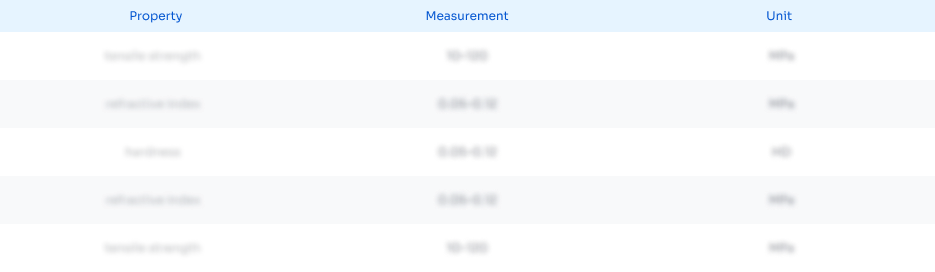
Abstract
Description
Claims
Application Information
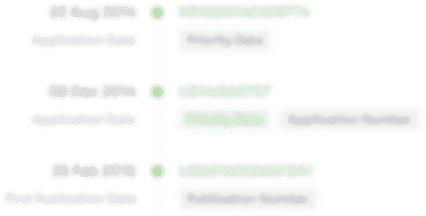
- R&D Engineer
- R&D Manager
- IP Professional
- Industry Leading Data Capabilities
- Powerful AI technology
- Patent DNA Extraction
Browse by: Latest US Patents, China's latest patents, Technical Efficacy Thesaurus, Application Domain, Technology Topic, Popular Technical Reports.
© 2024 PatSnap. All rights reserved.Legal|Privacy policy|Modern Slavery Act Transparency Statement|Sitemap|About US| Contact US: help@patsnap.com