Point Cloud Data Classification and Segmentation Method Based on Salient Point Sampling
A point cloud data, point sampling technology, applied in neural learning methods, character and pattern recognition, instruments, etc., can solve the problem of inability to extract local features of point cloud data, save parameters and computational overhead, reduce the amount of parameters, optimize The effect of convenience
- Summary
- Abstract
- Description
- Claims
- Application Information
AI Technical Summary
Problems solved by technology
Method used
Image
Examples
Embodiment Construction
[0078] The present invention will be further described below in conjunction with specific examples.
[0079] This embodiment provides a point cloud data classification and segmentation method based on salient point sampling, and proposes a point cloud data classification and segmentation network based on salient point sampling. The two network backbones are composed of a new salient point sampling algorithm (SPS) and Multi-scale local salient feature extraction network (MS-LSFE) is constructed, and both SPS and MS-LSFE can be flexibly inserted into other networks to assist sampling or feature extraction. For the convenience of calling, this method encapsulates SPS and MS-LSFE to form a multi-scale salient feature extraction module (MS-SFE), which achieves a balance between performance and parameter quantity. Such as figure 1 As shown, it shows our overall network structure (the top is the classification network, and the bottom is the segmentation network). In the figure, n is...
PUM
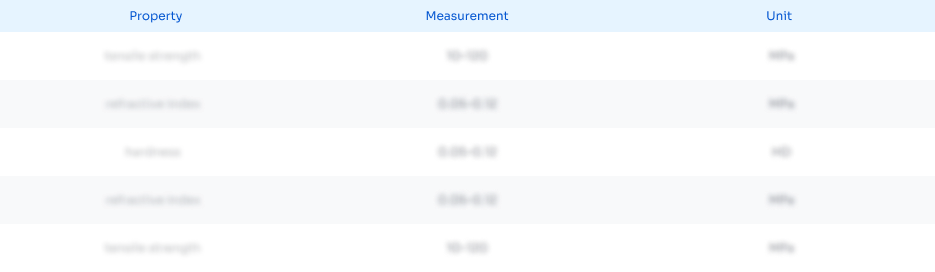
Abstract
Description
Claims
Application Information
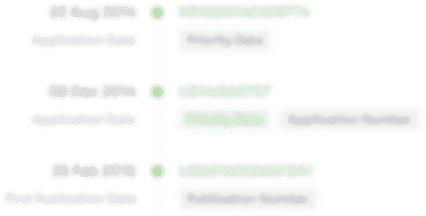
- R&D Engineer
- R&D Manager
- IP Professional
- Industry Leading Data Capabilities
- Powerful AI technology
- Patent DNA Extraction
Browse by: Latest US Patents, China's latest patents, Technical Efficacy Thesaurus, Application Domain, Technology Topic, Popular Technical Reports.
© 2024 PatSnap. All rights reserved.Legal|Privacy policy|Modern Slavery Act Transparency Statement|Sitemap|About US| Contact US: help@patsnap.com