Generation type dialogue abstracting method integrated with common knowledge
A generative and knowledge-based technology, applied in biological neural network models, natural language data processing, special data processing applications, etc., can solve problems such as low abstraction and inaccurate dialogue summaries
- Summary
- Abstract
- Description
- Claims
- Application Information
AI Technical Summary
Problems solved by technology
Method used
Image
Examples
specific Embodiment approach 1
[0032] Specific implementation mode 1: In this implementation mode, a generative dialogue summarization method incorporating common sense knowledge includes:
[0033] Step 1: Obtain the large-scale common sense knowledge base ConceptNet and the dialogue summary data set SAMSum.
[0034] Step 11. Obtain ConceptNet, a large-scale common sense knowledge base:
[0035] Get the large-scale common sense knowledge base ConceptNet from http: / / conceptnet.io / ; the common sense knowledge contained in it exists in the form of tuples, that is, tuple knowledge, which can be expressed as:
[0036] R = (h, r, t, w),
[0037] Among them, R represents a tuple knowledge; h represents the head entity; r represents the relationship; t represents the tail entity; w represents the weight, which represents the confidence of the relationship; It is w; for example R=(call, related, contact, 10), which means that the relationship between "call" and "contact" is "relevant", and the weight is 10; throug...
specific Embodiment approach 2
[0046] Embodiment 2: This embodiment differs from Embodiment 1 in that the step 2 utilizes the obtained large-scale common sense knowledge base ConceptNet to introduce tuple knowledge into the dialog summary data set SAMSum, and construct a heterogeneous dialog graph; specifically The process is:
[0047] Step 21. Obtain relevant knowledge of the dialogue; for a section of dialogue, the present invention first obtains a series of related tuple knowledge from ConceptNet according to the words in the dialogue, eliminates noise knowledge, and finally can obtain the tuple knowledge set relevant to the given dialogue, like Figure 4 ;
[0048] Step 22. Construct the sentence-knowledge map:
[0049] For the relevant tuple knowledge obtained in step 21, suppose there are sentence A and sentence B, word a belongs to sentence A, word b belongs to sentence B, if the tail entity h of the related knowledge of a and b is consistent, then sentence A Connect with sentence B to tail entity...
specific Embodiment approach 3
[0058] Embodiment 3: The difference between this embodiment and Embodiment 1 or 2 is that the step 31 is to construct a node encoder, and use a bidirectional long-short-time neural network (Bi-LSTM) to obtain node initialization representation and word initialization representation The specific process is:
[0059] For the heterogeneous dialogue graph proposed by the present invention in step 2, each node v i contains|v i | words, the sequence of words is where w i,n represents the node v i The nth word of n∈[1,|v i |]; use bidirectional long-short-time neural network (Bi-LSTM) to sequence words Generate forward hidden sequence and backward hidden sequence Among them, the forward hidden layer state backward hidden state x n means w i,n The word vector representation; the initial representation of the node is obtained by splicing the last hidden layer representation of the forward hidden layer state and the first hidden layer representation of the backward...
PUM
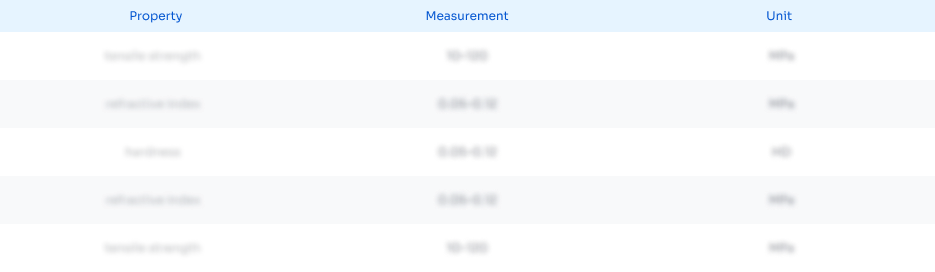
Abstract
Description
Claims
Application Information
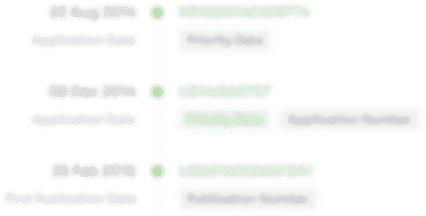
- R&D Engineer
- R&D Manager
- IP Professional
- Industry Leading Data Capabilities
- Powerful AI technology
- Patent DNA Extraction
Browse by: Latest US Patents, China's latest patents, Technical Efficacy Thesaurus, Application Domain, Technology Topic, Popular Technical Reports.
© 2024 PatSnap. All rights reserved.Legal|Privacy policy|Modern Slavery Act Transparency Statement|Sitemap|About US| Contact US: help@patsnap.com