Waveform optimization method of mimo radar based on iterative optimization network
A radar waveform, iterative optimization technology, applied in the radar field, can solve the problems of insensitivity, deep learning waveform design cannot converge initial value, etc., achieve excellent performance, superior performance, and solve the effect of invalid iteration
- Summary
- Abstract
- Description
- Claims
- Application Information
AI Technical Summary
Problems solved by technology
Method used
Image
Examples
Embodiment 1
[0111] MIMO radar consists of M antennas, each of which transmits N sub-pulse waveforms. In order to ensure the efficient use of energy, the signal is defined as a constant modulus, then each sub-pulse can be expressed as:
[0112]
[0113] where m=1,...,M, n=1,...,N. and need to guarantee y m (n) ∈ [0, 2π]. definition As the sequence of waveforms transmitted by the mth antenna. The formula (1) represents the nth sub-pulse signal transmitted by the mth antenna. Therefore, all sub-pulse waveform sets transmitted by all antennas can be represented as a matrix Since there are M signals, each of which transmits N sub-pulses, there are N rows and M columns in total. Each column represents the waveform emitted by an antenna, and each element represents a sub-pulse. Similarly, the phase matrix corresponding to the signal matrix can be expressed as
[0114] The orthogonality of waveforms is often determined by autocorrelation and cross-correlation. where the waveform ...
Embodiment 2
[0219] The two methods of the present invention are compared with the existing scheme "Document "H. He, P. Stoica, and J. Li, "Designing unimodular sequence sets with good correlations; including an application to MIMO radar," IEEE Trans.Signal Process., vol .57, no.11, pp.4391–4405, Nov. 2009, "the scheme published", the existing scheme two "document "Cui G, Yu X, Piezzo M, et al. Constant modulus sequenceset design with good correlation properties[ J].Signal Processing, 2017, 139:75-85. "Published Scheme" for comparison.
[0220] In this embodiment, the convergence factor θ of the outer iteration 1 =0.001, default input phase y 0 is a random normalized phase sequence. Convergence factor θ for inner iteration 2 =0.001, the maximum number of iterations of the inner loop N max = 5000, the minimum number of iterations N min =1000, the convergence interval is E=100, the default weight vector is l 1 =l 2 =l 3 =l 4 =l 5 =1. The learning rate of the Adam deep learning alg...
PUM
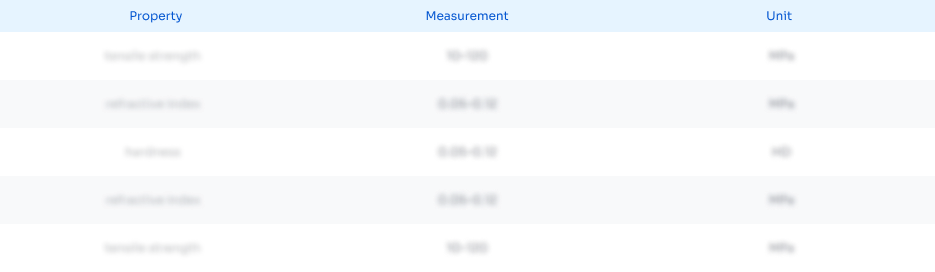
Abstract
Description
Claims
Application Information
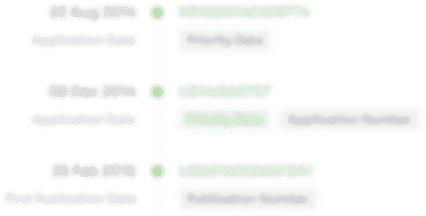
- Generate Ideas
- Intellectual Property
- Life Sciences
- Materials
- Tech Scout
- Unparalleled Data Quality
- Higher Quality Content
- 60% Fewer Hallucinations
Browse by: Latest US Patents, China's latest patents, Technical Efficacy Thesaurus, Application Domain, Technology Topic, Popular Technical Reports.
© 2025 PatSnap. All rights reserved.Legal|Privacy policy|Modern Slavery Act Transparency Statement|Sitemap|About US| Contact US: help@patsnap.com