A Multi-RGB-D Full Face Material Restoration Method Based on Deep Learning
A technology of deep learning and restoration method, which is applied in the field of 3D face reconstruction, can solve problems such as the lack of standardization of data sets and texture data, the inability to cover the expression of the side and rear of the human head, and the difficulty of texture image material restoration, etc., to expand the data range, Improve the effect of optimization and strong practicability
- Summary
- Abstract
- Description
- Claims
- Application Information
AI Technical Summary
Problems solved by technology
Method used
Image
Examples
Embodiment 1
[0077] The inventor tested the effectiveness of the differentiable rendering optimization module in step 2 in a simulation data set. Such as Figure 8 Figure (A) is the original image, Figure (B) is the schematic diagram of the 0th iteration, Figure (C) is the schematic diagram of the 10th iteration, and Figure (D) is the schematic diagram of the 150th iteration. As the number of iterations increases, the optimized material data is closer to the standard value than the initial results obtained using only the material estimation module.
Embodiment 2
[0079] The inventor tested the effectiveness of the differentiable rendering optimization module in step 2 for improving the loss function in the simulation data set. Figure 9 The test situation of a group of samples is shown, in which, picture (A) is a schematic diagram of the input image, picture (B) is a schematic diagram of the rendering result before improvement, picture (C) is a schematic diagram of the rendering result after improvement, and picture (D) is The albedo standard map, picture (E) is a schematic diagram of the albedo result before improvement, and picture (F) is a schematic diagram of the albedo result after improvement. It can be seen that before the loss function is improved, the error of the rendering result is small, but the error of restoring the texture is large. After the loss function is improved, the error of the rendering result is almost unchanged, and the restoration effect of the albedo texture is significantly improved.
Embodiment 3
[0081] The inventors tested the effectiveness of the inventive method on real samples. Such as Figure 10 Shown is the comparison diagram of the material restoration effect of the present invention in the actual sample test, wherein, Figure (A) is a schematic diagram of collecting photos, Figure (B) is a texture image synthesized by equipment, and Figure (C) is an optimized rendering result figure, and Figure (B) is a graph of optimized rendering results. (D) is a schematic diagram of putting back the original image for comparison. This method can restore the full face texture range including ears and neck, and the restored material data has high fidelity.
PUM
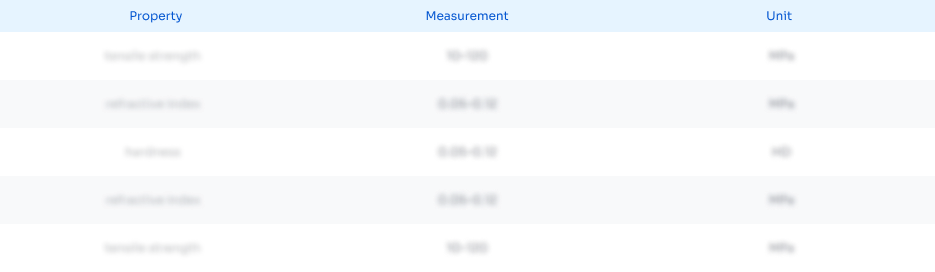
Abstract
Description
Claims
Application Information
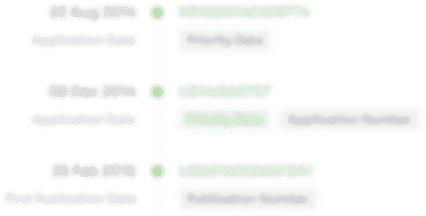
- R&D Engineer
- R&D Manager
- IP Professional
- Industry Leading Data Capabilities
- Powerful AI technology
- Patent DNA Extraction
Browse by: Latest US Patents, China's latest patents, Technical Efficacy Thesaurus, Application Domain, Technology Topic, Popular Technical Reports.
© 2024 PatSnap. All rights reserved.Legal|Privacy policy|Modern Slavery Act Transparency Statement|Sitemap|About US| Contact US: help@patsnap.com