Lane changing identification and prediction method, system and device for extracting vehicle trajectory by using roadside laser radar data, and storage medium
A technology of laser radar and vehicle trajectory, applied in the field of traffic engineering
- Summary
- Abstract
- Description
- Claims
- Application Information
AI Technical Summary
Problems solved by technology
Method used
Image
Examples
Embodiment 1
[0133] A lane change recognition and prediction method using roadside lidar data to extract vehicle trajectories, such as figure 1 shown, including the following steps:
[0134] (1) Background filtering and target clustering: use the background filtering technology to filter the background of the point cloud image obtained by the roadside lidar, and use the DBSCAN algorithm to cluster the point cloud in the point cloud image after the background filtering process , get the point cloud cluster;
[0135] (2) Object classification: distinguish pedestrians from vehicles, remove the point cloud of pedestrians, and only keep the point cloud of vehicles;
[0136] (3) Target tracking: generate lane boundary lines and match vehicles in transit to corresponding lanes;
[0137] (4) Lane-changing behavior prediction: Use the preset CDNLB and CCDNLB to predict the lane-changing behavior of the vehicle in real time.
Embodiment 2
[0139] According to a method of lane change recognition and prediction using roadside lidar data to extract vehicle trajectories described in Embodiment 1, the difference is that:
[0140] In step (1), background filtering refers to: filter out the background point cloud when no target in transit passes through from the point cloud image when the target in transit passes, and obtain the background point cloud of the target in transit; Figure 4 is the schematic diagram of point cloud data before background filtering; Figure 5 Is the schematic diagram of point cloud data after background filtering.
[0141] In step (1), target clustering includes the following steps:
[0142] A. Scan the entire point cloud image obtained after the background filtering process, find any core point, and expand the core point, that is, find all the density-connected data points starting from the core point;
[0143] B. Traverse all core points in the ε neighborhood of the core point (note: the ...
Embodiment 3
[0213] A lane change recognition and prediction system that uses roadside lidar data to extract vehicle trajectories, such as Figure 11 As shown, the lane change recognition and prediction method for extracting vehicle trajectories using roadside lidar data includes: a target point cloud collection module, a data processing module, and a prediction module; the target point cloud collection module is used to: collect in-transit targets, Background point cloud data; the data processing module is used to: filter out background point cloud data, filter out pedestrian point cloud data, cluster vehicle point clouds, track vehicle targets in different frames, divide lanes and match vehicle targets to Go in the corresponding lane; the prediction module is used to: predict whether the vehicle on the way will change lanes. The data processing module integrates a variety of point cloud processing algorithms: the DBSCAN algorithm is used for the clustering of target point clouds in trans...
PUM
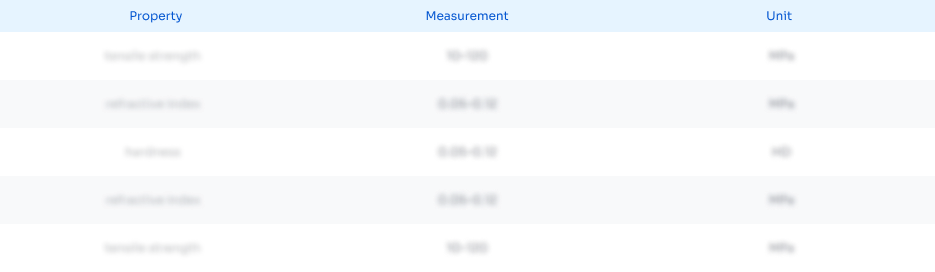
Abstract
Description
Claims
Application Information
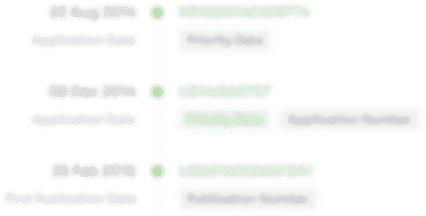
- R&D Engineer
- R&D Manager
- IP Professional
- Industry Leading Data Capabilities
- Powerful AI technology
- Patent DNA Extraction
Browse by: Latest US Patents, China's latest patents, Technical Efficacy Thesaurus, Application Domain, Technology Topic.
© 2024 PatSnap. All rights reserved.Legal|Privacy policy|Modern Slavery Act Transparency Statement|Sitemap