Software Failure Time Prediction Method Based on Correlation Vector Regression Estimation
A regression estimation and failure time technology, applied in the field of software failure time data prediction, which can solve problems such as over-learning applicability.
- Summary
- Abstract
- Description
- Claims
- Application Information
AI Technical Summary
Problems solved by technology
Method used
Image
Examples
Embodiment Construction
[0036] 1) Data normalization
[0037] When using the regression estimation algorithm for learning prediction, it is first necessary to normalize all input and output data to the interval [0.1,0.9]. The specific conversion formula is: y = 0.8 Δ x + ( 0.9 - 0.8 × x m a x Δ ) , Among them, y is the normalized value, x is the actual value, and x max is the maximum value in the data set, x min is the minimum value, Δ=x max -x min , after the forecast is over, the following mapping is used to map the data back to the actual value:
[0038] 2) Problem Transformation
[0039] Assume that the software failure time that has occurred is t 1 ,t 2 ,...,t n , let t l =...
PUM
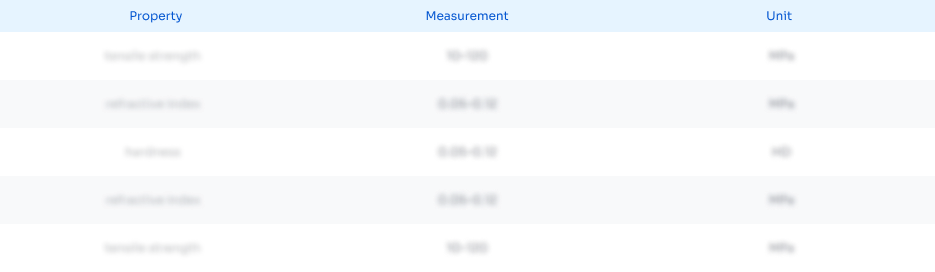
Abstract
Description
Claims
Application Information
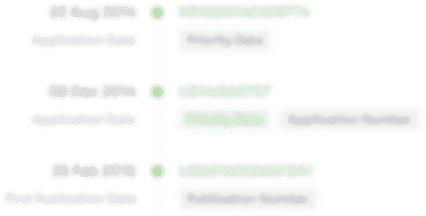
- Generate Ideas
- Intellectual Property
- Life Sciences
- Materials
- Tech Scout
- Unparalleled Data Quality
- Higher Quality Content
- 60% Fewer Hallucinations
Browse by: Latest US Patents, China's latest patents, Technical Efficacy Thesaurus, Application Domain, Technology Topic, Popular Technical Reports.
© 2025 PatSnap. All rights reserved.Legal|Privacy policy|Modern Slavery Act Transparency Statement|Sitemap|About US| Contact US: help@patsnap.com