Handwritten numeral recognition method and system
A digital recognition and handwriting technology, applied in the field of digital recognition, can solve the problems of inaccurate and single handwritten digital recognition
- Summary
- Abstract
- Description
- Claims
- Application Information
AI Technical Summary
Problems solved by technology
Method used
Image
Examples
Embodiment 1
[0071] figure 1 This is a flowchart of a method for recognizing handwritten digits provided in Embodiment 1 of the present application.
[0072] like figure 1 As shown, the method includes:
[0073] S101. Receive a handwritten digital image input by a user.
[0074] In this embodiment of the present application, the user can input handwritten numbers on the touch screen of a tablet or a mobile phone by handwriting, and the method first receives an image of the handwritten numbers input by the user on the touch screen of a mobile phone or a tablet or other tool.
[0075] S102, extracting M covariance features of the handwritten digital image.
[0076] In this embodiment of the present application, the user may input at least one training image in advance, and extract M types of covariance features for each training image respectively, the number of covariances extracted for each training image is the same, and multiple training images There is a one-to-one correspondence be...
Embodiment 2
[0082] figure 2 A flowchart of a method for recognizing handwritten digits provided in Embodiment 2 of the present application.
[0083] like figure 2 As shown, the method includes:
[0084] S201. Receive a handwritten digital image input by a user.
[0085] S202, extracting M covariance features of the handwritten digital image.
[0086] The execution process of steps S201-S202 provided in the second embodiment of this application is the same as the execution process of steps S101-S102 provided in the first embodiment. For the detailed description of the steps S201-S202 provided in the second embodiment of this application, please refer to this embodiment of the application Steps S101-S102 in Step 1 will not be repeated here.
[0087] S203, respectively calculating the distance between each covariance feature of the handwritten digital image and the covariance feature of each preset training image corresponding to each covariance feature of the handwritten digital image...
Embodiment 3
[0110] Figure 4 The third embodiment of the present application provides a flow chart of a method for setting a process of preset M covariance features of each training image.
[0111] like Figure 4 As shown, the method includes:
[0112] S401. Receive at least one training image input by a user.
[0113] In this embodiment of the present application, at least one training image input by the user may be received in advance.
[0114] S402: Extract M covariance features of each training image respectively.
[0115] In this embodiment of the present application, the user may input at least one training image in advance, and extract M types of covariance features for each training image respectively, the number of covariances extracted for each training image is the same, and multiple training images There is a one-to-one correspondence between the extraction methods of M covariances of each training image in That is to say, 4 covariance features are extracted for each trai...
PUM
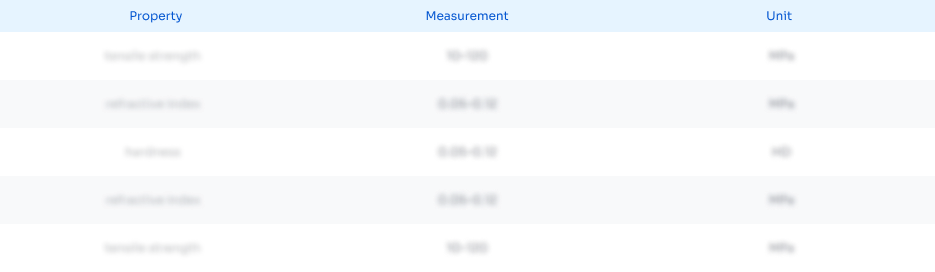
Abstract
Description
Claims
Application Information
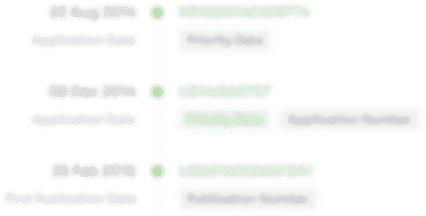
- R&D
- Intellectual Property
- Life Sciences
- Materials
- Tech Scout
- Unparalleled Data Quality
- Higher Quality Content
- 60% Fewer Hallucinations
Browse by: Latest US Patents, China's latest patents, Technical Efficacy Thesaurus, Application Domain, Technology Topic, Popular Technical Reports.
© 2025 PatSnap. All rights reserved.Legal|Privacy policy|Modern Slavery Act Transparency Statement|Sitemap|About US| Contact US: help@patsnap.com