A face beauty prediction method based on deep self-learning
A prediction method and self-learning technology, applied to instruments, character and pattern recognition, computer components, etc., can solve problems such as difficulties in research work, difficulty in finding extremely beautiful and extremely ugly images, and achieve a high degree of consistency
- Summary
- Abstract
- Description
- Claims
- Application Information
AI Technical Summary
Problems solved by technology
Method used
Image
Examples
Embodiment Construction
[0034] refer to Figure 1 to Figure 3 , a kind of face beauty prediction method based on depth self-learning of the present invention, comprises the following steps:
[0035] (1) Extract the LBP texture features of training face images, test face images and natural images;
[0036] (2) Based on the CDBN learning model, the LBP texture feature of the natural image is used as input, and the first layer of the CDBN is self-learned;
[0037] (3) The LBP texture feature of the training face image is used as an input, and the CDBN is greedy and unsupervised training layer by layer, and learns to represent the apparent features of the beautiful image of the face image;
[0038] (4) Utilize the CDBN that has completed the training to extract the training face image for beautiful prediction, the appearance feature of testing the face image;
[0039] (5) Use SVM regression to predict the beauty of the face image, and output the discrimination result.
[0040] The CDBN (Convolutional ...
PUM
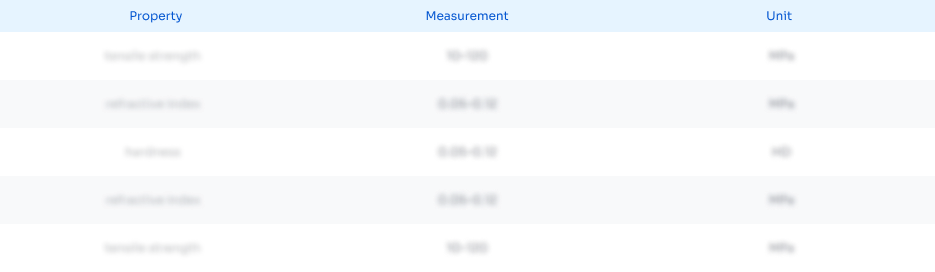
Abstract
Description
Claims
Application Information
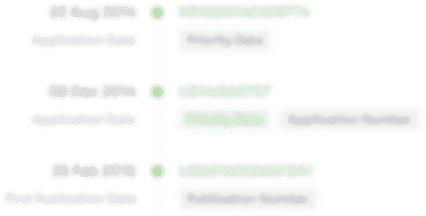
- R&D Engineer
- R&D Manager
- IP Professional
- Industry Leading Data Capabilities
- Powerful AI technology
- Patent DNA Extraction
Browse by: Latest US Patents, China's latest patents, Technical Efficacy Thesaurus, Application Domain, Technology Topic, Popular Technical Reports.
© 2024 PatSnap. All rights reserved.Legal|Privacy policy|Modern Slavery Act Transparency Statement|Sitemap|About US| Contact US: help@patsnap.com