Particle optimizing probability hypothesis density multi-target tracking method based on variation filtering
A probabilistic hypothesis density, multi-target tracking technology, applied in the field of multi-target tracking, can solve the problems of increased computational complexity and difficulty in engineering applications, and achieve the effect of improving the effect of state estimation
- Summary
- Abstract
- Description
- Claims
- Application Information
AI Technical Summary
Problems solved by technology
Method used
Image
Examples
Embodiment Construction
[0021] The present invention will be further described in detail below in conjunction with accompanying drawings and examples.
[0022] Under the framework of random set theory, the PHD filtering algorithm takes the state value set of all targets at the current moment as a state RFS variable, and the observation value set at the current moment as an observation RFS variable, and puts the multi-target tracking problem in the Bayesian It is solved under the framework of Siss filter. The only difference from the Bayesian optimal filter is that the Bayesian filter transfers the posterior probability density function of a single target state, while the PHD filter transfers the PHD of the multi-target state, that is, a part of the multi-target posterior density. step moment. figure 1 It shows the realization principle under the Monte Carlo method, that is, the particle PHD filter principle, D in the figure k-1 ( ) and D k (·) represent the PHD function of the target at time k-1 a...
PUM
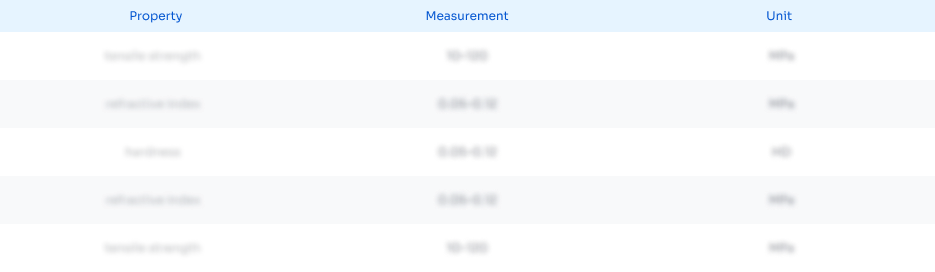
Abstract
Description
Claims
Application Information
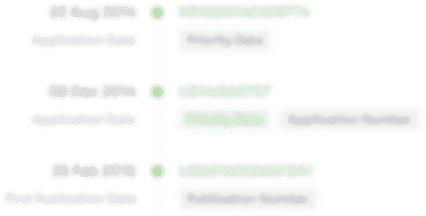
- R&D Engineer
- R&D Manager
- IP Professional
- Industry Leading Data Capabilities
- Powerful AI technology
- Patent DNA Extraction
Browse by: Latest US Patents, China's latest patents, Technical Efficacy Thesaurus, Application Domain, Technology Topic.
© 2024 PatSnap. All rights reserved.Legal|Privacy policy|Modern Slavery Act Transparency Statement|Sitemap