Blind source separation method
A technology of blind source separation and separation matrix, applied in the field of signal processing, to achieve the effect of improving diversity, reducing time complexity, and shortening search time
- Summary
- Abstract
- Description
- Claims
- Application Information
AI Technical Summary
Problems solved by technology
Method used
Image
Examples
Embodiment 1
[0027] Embodiment 1, the mathematical model of the present invention.
[0028] refer to figure 1 , a kind of blind source separation method of the present invention, the mathematical modeling in the present embodiment is: be provided with n source signals, they form n-dimensional vector: S (t)=[s 1 ,s 2 ,...,s n ] T ,s i ∈C n for mutual statistics independent The source signal; mutual statistics between vectors independent , m mixed signals constitute an m-dimensional observation data vector: X(t)=[x 1 ,x 2 ,...,x m ] T , x i ∈C m is the actual observed signal; the mathematical model of blind signal separation with noise is:
[0029] X(t)=A·S(t)+n(t)(1)
[0030] In the formula, A is an m×n dimensional mixing matrix, and n(t) is an m×n dimensional additive noise.
[0031] The solution to the blind source separation problem is to find a separation matrix W while ignoring the noise n(t), so that the separated matrix Y(t) satisfies:
[0032]
[0033] Here kur...
Embodiment 2
[0036] Embodiment 2, overall steps of the present invention.
[0037] refer to figure 2 , a blind source separation method of the present invention, in the present embodiment, the overall steps of the present invention are:
[0038] Step 1: Preprocessing the observed signal.
[0039] The preprocessing includes centering and whitening the mixed signal, and centering is also called mean removal, which can be realized by the following formula:
[0040]
[0041] The whitening operation on the mixed signal is irrelevant between the signals, and the pre-whitening of the random vector x is through the whitening matrix T, which has so that the transformed vector The correlation matrix satisfies Is an identity matrix I, the second-order statistics between components after whitening independent .
[0042] Step 2: Initialize the particle swarm. Use the gradient formula to generate particle swarms, and initialize the parameters of particle swarms, including c 1 and c 2 T...
Embodiment 3
[0049] Embodiment 3, the steps of the chaotic particle swarm algorithm of the present invention.
[0050] refer to image 3 , a blind source separation method of the present invention, in the present embodiment, the specific steps of the chaotic particle swarm algorithm of the present invention are:
[0051] Step 1: Read the information of the part of the particle swarm assigned as excellent particles, which includes the initial position of each particle, the initial velocity, the initial parameter value of the particle swarm and the fitness of each particle.
[0052] Step 2: Adaptively generate learning factor c through the following formula 1 、c 2 and inertial weights ω,
[0053]
[0054] c 1 (t)=2.5-2*exp(-α|f avg -f g |)(6)
[0055] c 2 (t)=0.5+2*exp(-α|f avg -f g |)(7)
[0056] In the formula, iter max is the maximum number of evolutions. f g is the optimal fitness, f avg is the average fitness of the particle swarm, and α is the control coefficient. ...
PUM
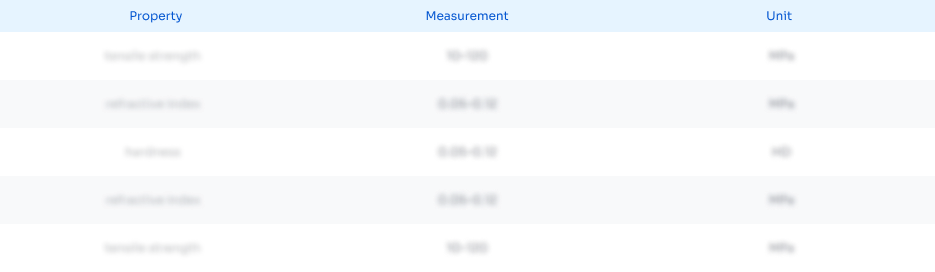
Abstract
Description
Claims
Application Information
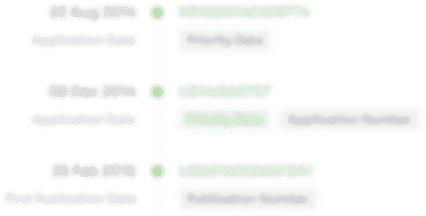
- R&D Engineer
- R&D Manager
- IP Professional
- Industry Leading Data Capabilities
- Powerful AI technology
- Patent DNA Extraction
Browse by: Latest US Patents, China's latest patents, Technical Efficacy Thesaurus, Application Domain, Technology Topic.
© 2024 PatSnap. All rights reserved.Legal|Privacy policy|Modern Slavery Act Transparency Statement|Sitemap