Improved fuzzy neural network bus intelligent scheduling method based on chaos theory
A fuzzy neural network and intelligent scheduling technology, applied in the field of intelligent transportation, can solve the problem that the local optimal solution of the particle swarm algorithm is easy to diverge, and achieve the effect of good convergence characteristics
- Summary
- Abstract
- Description
- Claims
- Application Information
AI Technical Summary
Problems solved by technology
Method used
Image
Examples
Embodiment 1
[0071] The system flow of bus intelligent dispatching is as follows: figure 1 shown. Specific steps are as follows:
[0072] The first step is the analysis and investigation of the actual problem demand. Under the relevant assumptions, the problem is described as follows: It is known that there are a total of J stations on a bus route with a total mileage of L. The operating time of the bus company’s vehicles in a day is [t 早 , t 晚 ], and the operating time can be divided into K periods, and the departure interval of the Kth period is Δt k. Assume that the buses for this route are of the same model and arrive at all stops on time. Passengers at each station are uniformly distributed, and the bus fare for each passenger is n. Starting from the operation profit of the bus company and the service level of the bus company (passengers’ waiting time is the shortest), consider the passenger flow and operating conditions of each station in a day, and hope to obtain the best vehi...
Embodiment 2
[0124] On the basis of Embodiment 1, it is further improved to increase the consideration of inertia factors and constraint factors, such as image 3 As shown, in order to keep the particle's motion inertia, it has the tendency to expand the search space and has the ability to explore new regions. However, the inertia weight w of the PSO algorithm with fixed parameters is usually less than 1, and the speed of the particles will become smaller and smaller, or even stop moving, and premature convergence will occur. The shrinkage factor method controls the final convergence of the system behavior, and can effectively search different regions, and the method can obtain high-quality solutions. Combining these two improved strategies together forms a particle swarm optimization algorithm with shrinkage factor and linearly decreasing inertia weight, which not only improves the convergence speed, but also avoids premature convergence.
[0125] Make the following improvements to formu...
Embodiment 3
[0131] Particle swarm optimization algorithm is an algorithm that starts from a group of initial solutions scattered in the solution space, which makes particle swarm optimization algorithm have good global convergence performance. Usually, when the randomly generated initial solutions are scattered in the solution space, the PSO algorithm can converge to the global optimal solution with a high probability. However, if the solution space is very large in practice, the random initial solution may only occupy a small corner, and the fly-operation often stagnates after finding a local optimal solution. This is related to the limited diversity of the particle swarm algorithm when the size of the particle swarm is small, which restricts the ability to jump out of the local optimal solution. Generally, we will increase the diversity by increasing the size of the particle swarm, so as to get rid of the limitation of the local optimal solution. But it often brings huge solution compl...
PUM
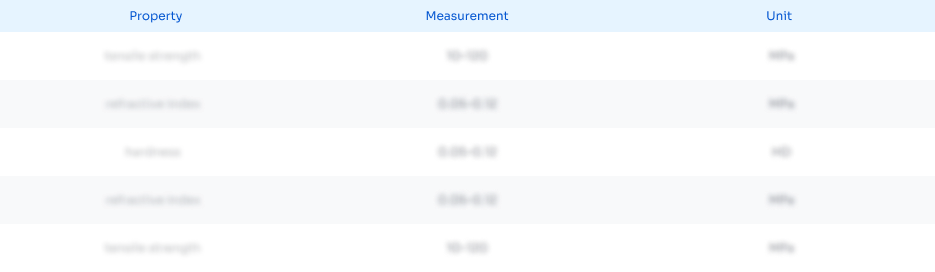
Abstract
Description
Claims
Application Information
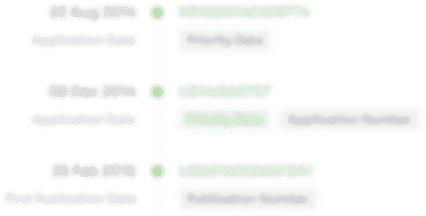
- R&D Engineer
- R&D Manager
- IP Professional
- Industry Leading Data Capabilities
- Powerful AI technology
- Patent DNA Extraction
Browse by: Latest US Patents, China's latest patents, Technical Efficacy Thesaurus, Application Domain, Technology Topic, Popular Technical Reports.
© 2024 PatSnap. All rights reserved.Legal|Privacy policy|Modern Slavery Act Transparency Statement|Sitemap|About US| Contact US: help@patsnap.com