High-dimensional data classification method based on two-stage mixed feature selection
A hybrid feature, high-dimensional data technology, applied in the fields of instruments, character and pattern recognition, computer components, etc., can solve the problems of easy to fall into local optimum, early convergence, easy overfitting of high-dimensional data, etc., to improve classification performance, improved operating speed, and the effect of accurate predictions
- Summary
- Abstract
- Description
- Claims
- Application Information
AI Technical Summary
Problems solved by technology
Method used
Image
Examples
Embodiment 1
[0087] Embodiment 1, high-dimensional data classification method based on two-stage mixed feature selection, such as Figure 1-5 As shown, firstly, the MIC method is used to obtain the correlation between features and labels, and then a suitable deletion threshold is learned according to the Q-Learning algorithm to obtain the selected feature subset; and then the improved Particle Swarm Optimization (PSO, Particle SwarmOptimization ) to search for the optimal feature subset, and then predict the label of the sample in the data set.
[0088] Step 1. Obtain the data set and process it;
[0089] Download the microarray data set from the Internet, then organize the characteristic information of the data in the host computer, mark the classification labels of all samples, and finally remove the serial number of each sample, delete the missing samples in the data set, and obtain the processed data set;
[0090] In this embodiment, 15 medical-related microarray data sets are obtaine...
PUM
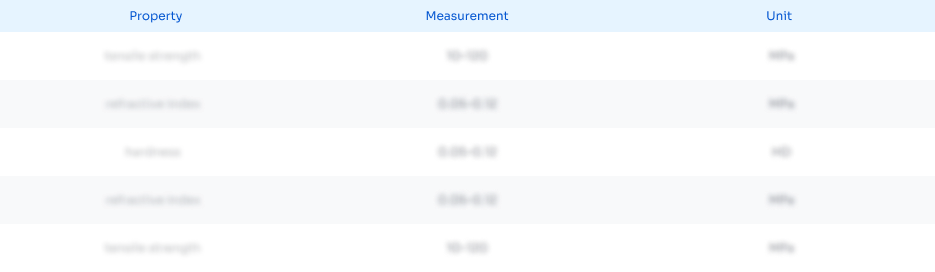
Abstract
Description
Claims
Application Information
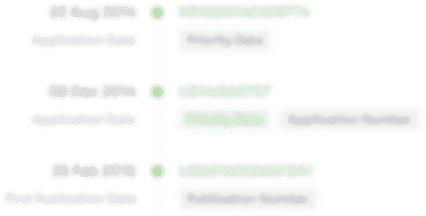
- R&D Engineer
- R&D Manager
- IP Professional
- Industry Leading Data Capabilities
- Powerful AI technology
- Patent DNA Extraction
Browse by: Latest US Patents, China's latest patents, Technical Efficacy Thesaurus, Application Domain, Technology Topic, Popular Technical Reports.
© 2024 PatSnap. All rights reserved.Legal|Privacy policy|Modern Slavery Act Transparency Statement|Sitemap|About US| Contact US: help@patsnap.com