Small-sample polarized SAR ground feature classification method based on deep convolutional twin network
A twin network and deep convolution technology, applied in the field of image processing, can solve the problems of reduced network classification accuracy, complex feature design, and high cost, and achieve the effect of improving classification performance and facilitating model classification.
- Summary
- Abstract
- Description
- Claims
- Application Information
AI Technical Summary
Problems solved by technology
Method used
Image
Examples
Embodiment Construction
[0031] The present invention will be introduced and described in detail below in conjunction with the accompanying drawings.
[0032] Refer to attached figure 1 , the specific implementation steps of the present invention are as follows:
[0033] Step 1. Input a polarimetric SAR image with a size of 430*280 to be classified and the real object label information corresponding to the polarimetric SAR image.
[0034] Step 2. Use the refined Lee filtering method to perform filtering processing on the polarimetric SAR image to be classified, remove the coherent speckle noise interference, and obtain the filtered polarimetric SAR image to be classified.
[0035] Step 3. Extracting the polarimetric SAR input feature vector from the filtered polarimetric SAR image to be classified.
[0036] (3a) Express each pixel in the polarimetric SAR image data with a 3*3 polarimetric coherence matrix T:
[0037]
[0038] (3b) Extract the polarization eigenvector I of the corresponding pixel...
PUM
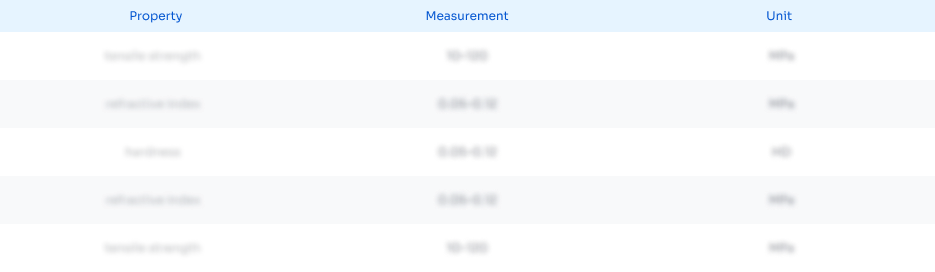
Abstract
Description
Claims
Application Information
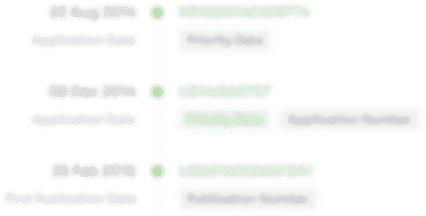
- R&D Engineer
- R&D Manager
- IP Professional
- Industry Leading Data Capabilities
- Powerful AI technology
- Patent DNA Extraction
Browse by: Latest US Patents, China's latest patents, Technical Efficacy Thesaurus, Application Domain, Technology Topic, Popular Technical Reports.
© 2024 PatSnap. All rights reserved.Legal|Privacy policy|Modern Slavery Act Transparency Statement|Sitemap|About US| Contact US: help@patsnap.com