Context sensing and complex semantic association based data space modeling method
A technology of semantic correlation and modeling method, which is applied in the fields of electronic digital data processing, special data processing applications, semi-structured data retrieval, etc., can solve the problems of low query accuracy and long average response time of keyword queries, etc., and achieve expression powerful effect
- Summary
- Abstract
- Description
- Claims
- Application Information
AI Technical Summary
Problems solved by technology
Method used
Image
Examples
specific Embodiment approach 1
[0022] Specific implementation mode one: combine Figure 11 To illustrate this embodiment, the data space modeling method based on context awareness and complex semantic association in this embodiment is specifically prepared according to the following steps:
[0023] Step 1. Construct a semi-structured graph model, called the context-aware complex semantic association network model COSAN; Step 2, represent the context-aware interpretation object according to the context-aware complex semantic association network model COSAN; Step 3, according to the context Perceived interpretation objects derive context-aware basic semantic associations and complex multiple semantic associations; step 4, derive semantic association inference rules based on context-aware basic semantic associations and complex multiple semantic associations.
[0024] We first propose a semi-structured graph model called the context-aware complex semantic association network model COSAN, then describe in detai...
specific Embodiment approach 2
[0025] Specific embodiment two: the difference between this embodiment and specific embodiment one is: a kind of semi-structured graph model is built in the described step one, is referred to as context-aware complex semantic association network model COSAN; Concrete process is:
[0026] To address the problems or challenges mentioned above, this subsection proposes a semi-structured graph model, called the COSAN model. In our model, the main considerations are as follows:
[0027] (4). Not only consider the unified representation of structured, semi-structured and unstructured data, but also consider the impact of context. Furthermore, context should also be considered when expressing simple binary semantic associations.
[0028] (5). The traditional data space model puts too much emphasis on the representation of entities, ignoring the representation of complex semantic relations between entities. Specifically, this chapter formalizes it through a set of constraint sets th...
specific Embodiment approach 3
[0038] Embodiment 3: The difference between this embodiment and Embodiment 1 or 2 is that in the second step, the context-aware complex semantic association network model COSAN represents context-aware heterogeneous data and complex semantic relationships; the specific process is:
[0039] 1. Context-aware heterogeneous data representation
[0040] This subsection first gives some definitions, aiming to formalize the description of context-aware interpretation objects; then, an example is used to demonstrate how to represent context-aware heterogeneous data.
[0041] Definition 2.1 Context Conditions.
[0042] A context condition c is dΔν where,
[0043] 1) d is the dimension name of the context condition c, for example, time;
[0044] 2) Δ is an operator, such as, =, ≠, ≤, , ≥, in, not in, between, between + , between - , and between*;
[0045] 3) ν is a value expression corresponding to dimension d, such as {b,...,e};
[0046] Assumption: if the operator is = or ≠, the...
PUM
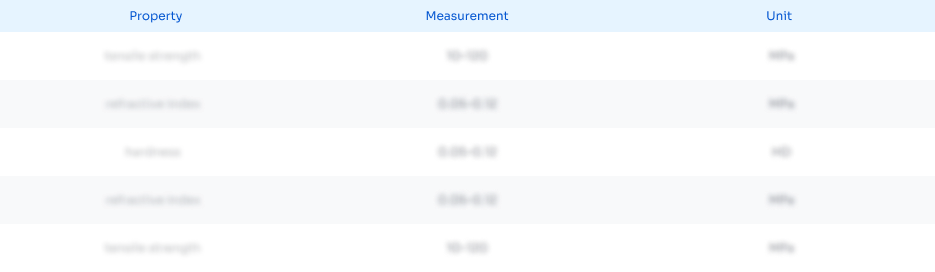
Abstract
Description
Claims
Application Information
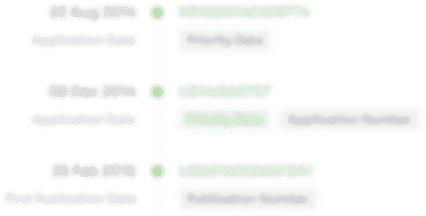
- R&D
- Intellectual Property
- Life Sciences
- Materials
- Tech Scout
- Unparalleled Data Quality
- Higher Quality Content
- 60% Fewer Hallucinations
Browse by: Latest US Patents, China's latest patents, Technical Efficacy Thesaurus, Application Domain, Technology Topic, Popular Technical Reports.
© 2025 PatSnap. All rights reserved.Legal|Privacy policy|Modern Slavery Act Transparency Statement|Sitemap|About US| Contact US: help@patsnap.com