Medical field-oriented named entity identifying method based on deep learning
A named entity recognition and deep learning technology, applied in the medical field, can solve the problem that the CRF model does not consider semantic information, meaningless annotation results, etc., to reduce the consumption of human and material resources and improve performance.
- Summary
- Abstract
- Description
- Claims
- Application Information
AI Technical Summary
Problems solved by technology
Method used
Image
Examples
specific Embodiment approach 1
[0038] Specific implementation mode one: combine figure 1 A named entity recognition method based on deep learning for the medical field of this embodiment is specifically prepared according to the following steps:
[0039] Step 1. Use unlabeled corpus for word vector vec i Training (using the word2vec toolkit for word vector training), the vocabulary voc of the supplementary medical field corpus (this vocabulary contains more vocabulary than the medical field corpus) and the word vector vec corresponding to the vocabulary voc ;where, i=1,2,3,...,n; vec=vec 1 ,vec 2 ,...,vec i ,...,vec n ;voc=voc 1 ,voc 2 ,...,voc i ,...,voc n ; n is the total number of word categories in the unlabeled corpus;
[0040] Step 2. Use the training corpus in the marked corpus to train the long-short-term memory unit network LSTM; use the word vector vec obtained in step 1 as the pre-training vector, and use the LSTM method according to the pre-training vector, x k and y k Calculate the o...
specific Embodiment approach 2
[0065] Specific implementation mode two: the difference between this implementation mode and specific implementation mode one is: the input sequence X of the LSTM neural network is calculated by using method one described in step two one. The specific process:
[0066] Establish the training corpus vocabulary voc' in the marked corpus, and merge voc' and voc into the vocabulary VOC; VOC=VOC 1 ,VOC 2 ,VOC 3 ,...,VOC N ;
[0067] Randomly initialize the vector matrix word_emb corresponding to the vocabulary VOC, so that the dimension of the vector matrix word_emb is the same as the word vector vec, and assign values according to formula (1):
[0068] w o r d _ emb i = vec i , ∀ i ∈ v o c - - - ( 1 ) ...
specific Embodiment approach 3
[0073] Specific implementation mode three: the difference between this implementation mode and specific implementation mode one or two is: the input sequence X of the LSTM neural network is obtained by calculating the input sequence X of the LSTM neural network as described in step two one:
[0074] Randomly initialize the vector matrix word_emb corresponding to the vocabulary VOC, and keep the vector word_emb after assignment according to formula (1) i Unchanged, that is, it is not updated as a parameter, and then the vector matrix corresponding to a vocabulary in the vocabulary VOC is randomly initialized as word_emb_para, and the input sequence X of the LSTM neural network is calculated:
[0075] X = ( x k [ k 1 , k 2 ] · w...
PUM
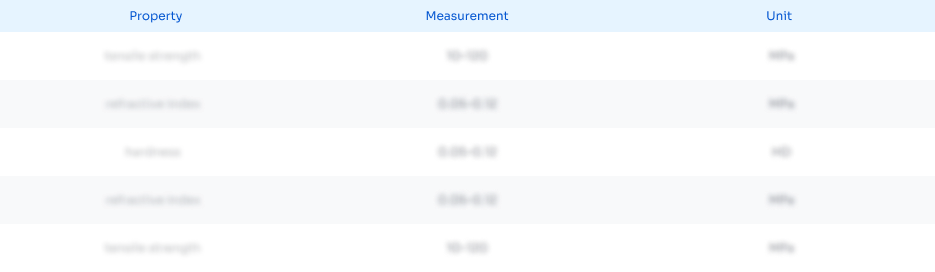
Abstract
Description
Claims
Application Information
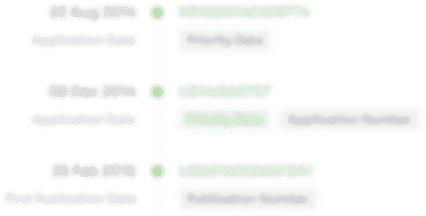
- R&D
- Intellectual Property
- Life Sciences
- Materials
- Tech Scout
- Unparalleled Data Quality
- Higher Quality Content
- 60% Fewer Hallucinations
Browse by: Latest US Patents, China's latest patents, Technical Efficacy Thesaurus, Application Domain, Technology Topic, Popular Technical Reports.
© 2025 PatSnap. All rights reserved.Legal|Privacy policy|Modern Slavery Act Transparency Statement|Sitemap|About US| Contact US: help@patsnap.com