A fast clustering method for commodity-related big data sparse network
A clustering method and big data technology, applied in the field of big data processing, can solve problems such as association analysis of big data not suitable for retail commodities, achieve flexible memory dynamic management, eliminate redundant space, and improve mining efficiency.
- Summary
- Abstract
- Description
- Claims
- Application Information
AI Technical Summary
Problems solved by technology
Method used
Image
Examples
Embodiment Construction
[0050] The following describes in detail the fast clustering method of commodity-related big data sparse network proposed by the present invention with reference to the accompanying drawings.
[0051] Such as figure 1 As shown, the present invention includes three clustering stages: (1) using a single-step linked list structure to store the common purchase relationship matrix of retail goods; (2) pruning the low-level commodity nodes of the commodity-related big data sparse network; 3) Use the idea of dividing high-value commodity nodes by low-value commodity nodes to quickly cluster commodity-related big data sparse networks.
[0052] The following specific examples illustrate the implementation process of the present invention. Assuming that there are 8 kinds of commodities, the sales records are as follows:
[0053] Sales records of 8 products
[0054]
[0055] Note: "√" in the form means that the product has been purchased.
[0056] Specific steps are as follows:
[0057] A. Pre...
PUM
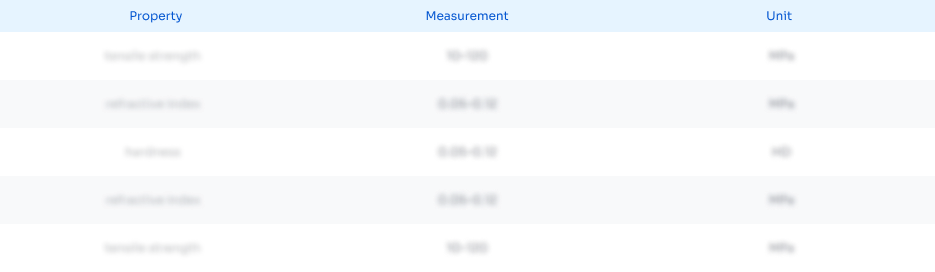
Abstract
Description
Claims
Application Information
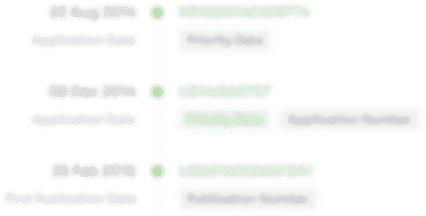
- R&D Engineer
- R&D Manager
- IP Professional
- Industry Leading Data Capabilities
- Powerful AI technology
- Patent DNA Extraction
Browse by: Latest US Patents, China's latest patents, Technical Efficacy Thesaurus, Application Domain, Technology Topic, Popular Technical Reports.
© 2024 PatSnap. All rights reserved.Legal|Privacy policy|Modern Slavery Act Transparency Statement|Sitemap|About US| Contact US: help@patsnap.com