Human face super-resolution algorithm based on regional depth convolution neural network
A deep convolution and super-resolution technology, applied in the field of face super-resolution algorithms, can solve the problems of not being able to make full use of the structural knowledge of face images, and the lack of expression ability of single-layer learning structures.
- Summary
- Abstract
- Description
- Claims
- Application Information
AI Technical Summary
Problems solved by technology
Method used
Image
Examples
Embodiment Construction
[0061] In order to make the object, technical solution and advantages of the present invention clearer, the present invention will be further described in detail below in conjunction with the accompanying drawings and embodiments. It should be understood that the specific embodiments described here are only used to explain the present invention, not to limit the present invention.
[0062] Such as figure 1 As shown, the face super-resolution algorithm based on the regional deep convolutional neural network of the embodiment of the present invention comprises the following steps:
[0063] Training phase:
[0064] S1. Obtain the trained high-resolution face image, process it to obtain a low-resolution face image, use the sliding window to adaptively select adjacent image blocks, and divide the low-resolution face image in the pixel domain to overlap each other The image block of , get multiple local regions;
[0065] The method to process the low-resolution face image is:
...
PUM
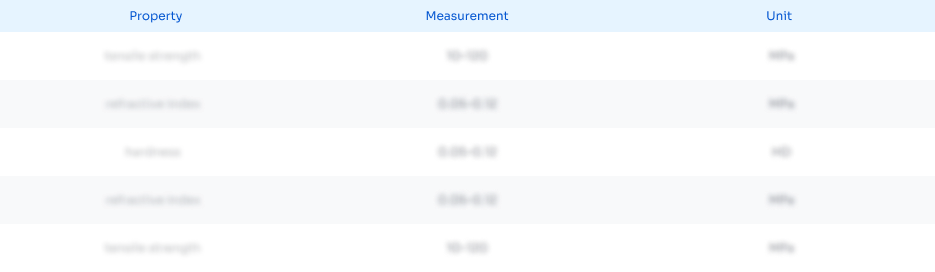
Abstract
Description
Claims
Application Information
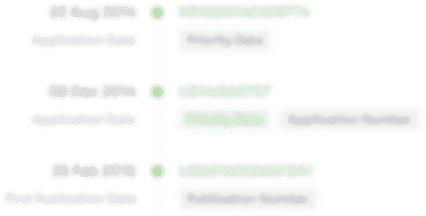
- R&D Engineer
- R&D Manager
- IP Professional
- Industry Leading Data Capabilities
- Powerful AI technology
- Patent DNA Extraction
Browse by: Latest US Patents, China's latest patents, Technical Efficacy Thesaurus, Application Domain, Technology Topic, Popular Technical Reports.
© 2024 PatSnap. All rights reserved.Legal|Privacy policy|Modern Slavery Act Transparency Statement|Sitemap|About US| Contact US: help@patsnap.com