Image object co-segmentation method guided by local shape migration
A technology of image object and co-segmentation, which is applied in the field of image processing and computer vision, can solve problems that affect the segmentation results, achieve high execution time and space efficiency, and solve the effect of poor segmentation results
- Summary
- Abstract
- Description
- Claims
- Application Information
AI Technical Summary
Problems solved by technology
Method used
Image
Examples
Embodiment Construction
[0036] Such as figure 1 As shown, the present invention proposes an image object co-segmentation method guided by local shape transfer, comprising the following steps:
[0037] (1) Image set preprocessing: Input M images containing objects of the same semantic category, use the saliency detection method proposed by Zhang et al. in 2015 to analyze the saliency of each image, and use the double mean of the saliency detection results Do the threshold to get the mask map, which is the initial segmentation result of the foreground and background, where the mask map is only composed of 0 and 1, 1 represents the foreground pixel point, and 0 represents the background pixel point.
[0038] (2) Perform dense feature point matching on any two images: for each image i (i=1,2,...,M), generate a 128-dimensional dense sift feature for each pixel on the image; The dense sift feature of picture i (i=1,2,...,M) and the dense sift feature of other pictures j (j=1,2,...,M and j≠i) adopt Kim et ...
PUM
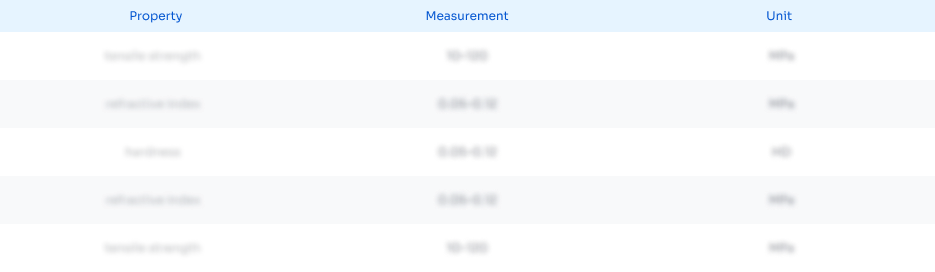
Abstract
Description
Claims
Application Information
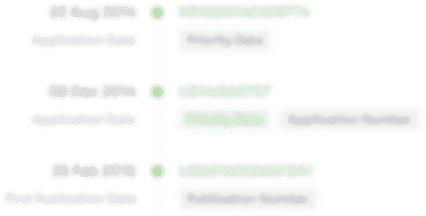
- R&D Engineer
- R&D Manager
- IP Professional
- Industry Leading Data Capabilities
- Powerful AI technology
- Patent DNA Extraction
Browse by: Latest US Patents, China's latest patents, Technical Efficacy Thesaurus, Application Domain, Technology Topic, Popular Technical Reports.
© 2024 PatSnap. All rights reserved.Legal|Privacy policy|Modern Slavery Act Transparency Statement|Sitemap|About US| Contact US: help@patsnap.com