Scene and target identification method and device based on multi-task learning
A multi-task learning and target recognition technology, applied in character and pattern recognition, instruments, biological neural network models, etc., can solve problems such as difficult and effective functions
- Summary
- Abstract
- Description
- Claims
- Application Information
AI Technical Summary
Problems solved by technology
Method used
Image
Examples
Embodiment 1
[0084] Such as figure 1 As shown, a method for scene and target recognition based on multi-task learning, the method includes the following steps:
[0085] Step S1: Collect pictures containing different scenes and objects as image sample data;
[0086] Step S2: Manually label the image sample data to obtain the target category label and the scene category label;
[0087] Step S3: Construct a multi-layer convolutional neural network model and perform network initialization;
[0088] Step S4: Using the image sample data and the corresponding target category labels, pre-train the constructed model until convergence to obtain the target recognition model;
[0089] Step S5: Based on the multi-task learning technology, add network branches to a specific layer of the target recognition model, and initialize randomly to obtain a multi-task network;
[0090] Step S6: Retrain the multi-task network by using image sample data and corresponding scene category labels and target category...
Embodiment 2
[0093] Such as figure 1 As shown, a method for scene and target recognition based on multi-task learning, the method includes the following steps:
[0094] Step S1: Collect pictures containing different scenes and objects as image sample data; including the following steps:
[0095] Step S11: an image collection step, using cameras and network resources to collect image data of different scenes and objects;
[0096] Step S12: an image screening step, performing secondary screening on the image data, removing image data with unsatisfactory picture quality and screen content, and using the image data of the remaining images as image sample data. Remaining images ≥ 3000. Preferably, there are more than 20,000 remaining images.
[0097] Step S2: Manually label the image sample data to obtain the target category label and the scene category label; including the following steps:
[0098] Step S21: mark the target category, mark N_ob target category labels for each image, and sto...
PUM
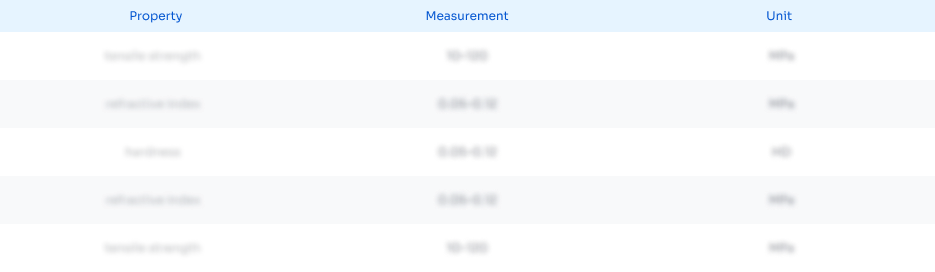
Abstract
Description
Claims
Application Information
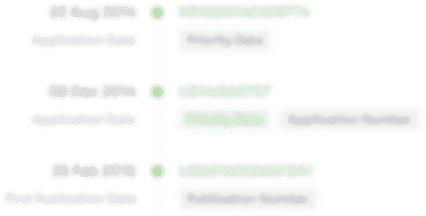
- R&D Engineer
- R&D Manager
- IP Professional
- Industry Leading Data Capabilities
- Powerful AI technology
- Patent DNA Extraction
Browse by: Latest US Patents, China's latest patents, Technical Efficacy Thesaurus, Application Domain, Technology Topic, Popular Technical Reports.
© 2024 PatSnap. All rights reserved.Legal|Privacy policy|Modern Slavery Act Transparency Statement|Sitemap|About US| Contact US: help@patsnap.com