Semi-supervised Gaussian process regression soft measurement modeling method improving self-training algorithm
A Gaussian process regression and modeling method technology, applied in the field of semi-supervised Gaussian process regression soft sensor modeling, can solve the problems of indistinguishable estimated values and missing leading variable sample estimates
- Summary
- Abstract
- Description
- Claims
- Application Information
AI Technical Summary
Problems solved by technology
Method used
Image
Examples
Embodiment Construction
[0014] Combine below figure 1 Shown, the present invention is described in further detail:
[0015] Take the common chemical process—debutanizer process as an example. The experimental data come from the debutanizer E process to predict the butane concentration.
[0016] Step 1: Collect a labeled sample set {X L ,Y L}, L means labeled; and unlabeled sample set {X U}, U means unlabeled, and for each sample x in the unlabeled sample set i ∈{X U}, calculate its relationship with each sample x in the labeled sample set j ∈{X L ,j=1,2,...,N L} similarity, N L Indicates the number of labeled samples, the calculation of the similarity index Sim is shown in formula (1), and the similarity in descending order is recorded as RSim, where γ∈(0,1) is the similarity parameter, ||x i -x j ||, cosi ,x j >respectively represent the vector x i ,x j The Euclidean distance between and the cosine of the included angle.
[0017] Sim j =γexp(-||x i -x j ||)+(1-γ)cosi ,x j > (1)
...
PUM
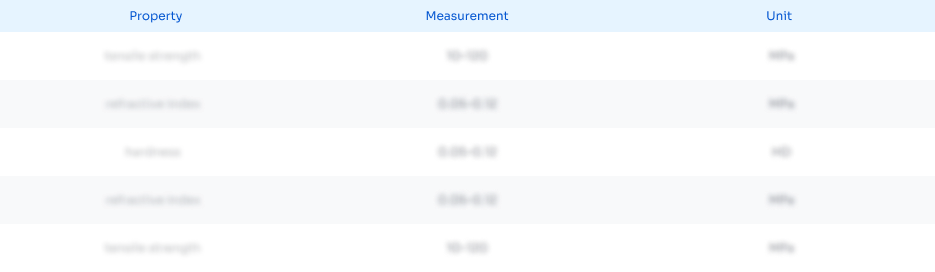
Abstract
Description
Claims
Application Information
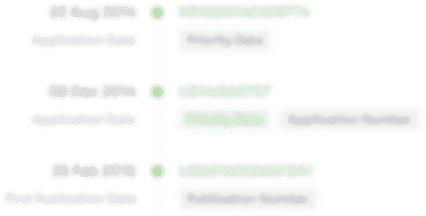
- R&D Engineer
- R&D Manager
- IP Professional
- Industry Leading Data Capabilities
- Powerful AI technology
- Patent DNA Extraction
Browse by: Latest US Patents, China's latest patents, Technical Efficacy Thesaurus, Application Domain, Technology Topic, Popular Technical Reports.
© 2024 PatSnap. All rights reserved.Legal|Privacy policy|Modern Slavery Act Transparency Statement|Sitemap|About US| Contact US: help@patsnap.com